What constitutes a central concept in an analysis, and how can it be effectively identified?
A central concept, or core idea, serves as the foundational element upon which an entire analysis is built. It is the primary subject of investigation, defining the scope and direction of the inquiry. Identifying this concept involves careful consideration of the overall subject matter, its key components, and the overarching goals of the analysis. Identifying this central concept is the starting point of any significant study.
The significance of this central concept lies in its ability to unify disparate elements within the subject matter, offering a cohesive framework for understanding complex issues. Effective identification of this central idea streamlines the analytical process, preventing unnecessary tangents and ensuring the study remains focused on the core objectives. A well-defined central concept promotes a comprehensive understanding, enabling analysts to draw meaningful conclusions and make accurate predictions.
Moving forward, a nuanced understanding of this central concept is crucial for analyzing the subsequent components and evaluating the overall analysis. Without a strong grounding in the central idea, conclusions risk becoming superficial and lacking in impact. The following sections will explore how various analytical methodologies can be applied to identify and interpret this core element within various contexts.
y_
Understanding the multifaceted nature of "y_" is essential for a comprehensive analysis. The following key aspects provide crucial insights into its various dimensions.
- Definition
- Application
- Context
- Measurement
- Interpretation
- Relationship
- Impact
- Comparison
The eight key aspectsdefinition, application, context, measurement, interpretation, relationship, impact, and comparisoncollectively illuminate the significance of "y_." For instance, the definition establishes a foundational understanding, while application demonstrates practical usage. Contextual analysis clarifies the specific situation in which "y_" plays a role. Measurement quantifies the phenomenon, facilitating comparisons with other variables, and understanding its impact on related outcomes. Comparative analysis highlights the differing influences of "y_" across various scenarios, providing insights into its potential relationships and wider implications. Without these interconnected facets, a thorough comprehension of "y_" remains incomplete.
- Amazing Bris Nails Stylish Designs Amp Expert Care
- Charming Alturas California Explore The High Sierra
1. Definition
A precise definition of "y_" is fundamental to any meaningful analysis. Without a clear understanding of its scope and characteristics, subsequent explorations risk becoming disconnected and unfocused. The definition serves as the bedrock upon which other aspects of "y_" are built, ensuring that all subsequent inquiries remain grounded in a shared, coherent understanding.
- Specificity and Scope
A rigorous definition must clearly delineate the parameters of "y_," avoiding ambiguity or vagueness. This involves specifying the key attributes, characteristics, and boundaries of the subject. For example, a definition of "economic growth" must specify whether it refers to GDP growth, per capita income, or a combination of factors. Failure to precisely define the scope can lead to misinterpretations and flawed analyses.
- Operationalization
A robust definition must be operationalizable, meaning it must offer a clear and measurable framework for assessing "y_" in practical contexts. This entails outlining specific indicators, metrics, or procedures to quantify or observe the phenomenon. For instance, defining "customer satisfaction" might involve surveys, feedback mechanisms, or quantifiable behavioral data. This operationalization process provides a framework for objective analysis.
- Contextual Application
The definition of "y_" must be sensitive to the specific context within which it is applied. A definition relevant to one field or domain might be inadequate or misleading in another. A definition of "success" for a startup might differ significantly from a definition relevant to the career progression of a long-term employee. Considering the specific context, and tailoring the definition to fit it, ensures accurate interpretation.
The precise definition of "y_" anchors all further analysis. By establishing clear boundaries, specifying operational measures, and considering contextual factors, the definition enables reliable and consistent evaluation of "y_" across diverse applications and situations. Without a robust definition, subsequent discussions on "y_" risk losing clarity and direction, ultimately hindering a coherent understanding of its role and influence.
2. Application
The application of "y_" encompasses the practical implementation and utilization of its principles, concepts, or elements. Its relevance stems from its direct impact on various fields and activities. Examining these applications provides critical insights into the practical significance of "y_" and its potential for use and impact.
- Practical Implementation
This facet encompasses the direct use of "y_" in real-world situations. Examples range from applying mathematical formulas to solve engineering problems to utilizing psychological principles to improve team performance. The effectiveness of this practical implementation depends heavily on the accuracy and precision of the understanding of "y_." Failures to apply "y_" correctly can result in inefficiencies, errors, or unintended consequences.
- Methodological Integration
The application of "y_" frequently involves integrating it into existing methodologies or frameworks. Consider, for example, integrating sustainability metrics into business models, or incorporating ethical considerations into software development. Successful integration relies on a deep understanding of both "y_" and the methodologies it complements, ensuring alignment and avoiding disruptions or conflicts.
- Problem-Solving Applications
"y_" often plays a crucial role in tackling challenges. Its successful application in problem-solving depends on recognizing the specific area where "y_" can be applied and tailoring its use to that context. Examples include using data analysis to identify trends in market behavior or employing scientific methods to develop new solutions for societal problems.
- Systemic Design Considerations
In many cases, "y_" is a key factor in designing complex systems. From creating efficient supply chains to designing user-friendly interfaces, "y_" informs decisions regarding system structure, organization, and workflow. Carefully considering the applications of "y_" during design ensures optimal system functioning and minimizes potential issues.
The diverse applications of "y_" highlight its broad applicability and importance across various fields. Understanding these practical applications not only illuminates the potential uses of "y_" but also emphasizes the significance of accurate understanding and thoughtful implementation for desired outcomes.
3. Context
The relationship between "Context" and "y_" is fundamental. Contextual understanding is indispensable for accurate interpretation and application of "y_." A concept's meaning is often deeply intertwined with the specific environment in which it's applied. This exploration examines key facets of this interplay, emphasizing the significance of context in comprehending "y_."
- Historical and Temporal Context
Historical context significantly shapes the understanding of "y_." Factors such as the prevailing societal norms, technological advancements, and political climate of a specific period profoundly influence the definition and meaning of "y_." For example, economic models developed in the pre-industrial era might not accurately reflect current economic realities. Similarly, interpretations of social trends need to be evaluated within the historical context in which they emerged.
- Geographical Context
Geographical context also plays a vital role in interpreting "y_." Cultural values, environmental factors, and resource availability within a specific region can significantly impact the application and interpretation of "y_." For instance, the effectiveness of a particular agricultural technique will vary depending on the climate and soil conditions of the region.
- Social and Cultural Context
Social and cultural norms exert a powerful influence on the understanding and application of "y_." Differing social structures, value systems, and cultural practices lead to varying interpretations of "y_." For example, the concept of success may be defined differently across cultures or socioeconomic groups.
- Technological Context
Technological advancements often reshape the landscape surrounding "y_." New technologies can alter the very nature of "y_," either by enhancing its application or by making certain applications obsolete. Modern communication technologies, for instance, have transformed how information is disseminated and the way interactions occur, impacting numerous aspects of "y_."
Considering the interconnectedness of historical, geographical, social, and technological contexts is crucial for comprehending "y_." A nuanced analysis must account for the dynamic interplay between these facets, recognizing that "y_" itself evolves alongside the contexts in which it is applied. This recognition emphasizes that understanding "y_" is not simply about defining it, but also about contextualizing it within a broader societal and environmental framework.
4. Measurement
Accurate measurement is indispensable for understanding and effectively analyzing "y_." Appropriate methodologies for quantifying "y_" provide a crucial foundation for drawing valid conclusions, forming reliable predictions, and establishing meaningful comparisons. The chosen metrics directly impact the interpretation and subsequent applications of knowledge derived from "y_." This section explores critical aspects of measurement in relation to "y_."
- Defining Measurable Units
A precise definition of the units used for measuring "y_" is paramount. Ambiguous or inconsistent definitions lead to inaccurate or misleading conclusions. Clear and universally agreed-upon units ensure comparability across different contexts and time periods, facilitating the identification of patterns and trends. Examples include standardized scales for assessing customer satisfaction or specific formulas for calculating economic growth. Lack of this crucial precision renders measurement unreliable and hampers the ability to draw conclusive insights from the data.
- Choosing Appropriate Metrics
The selection of relevant metrics is critical. Metrics must effectively capture the nuances of "y_" within its specific context. Inadequate metrics fail to provide an accurate representation, producing misleading results. The choice must align with the goals of the analysis and ensure the data encompasses all the factors necessary to understand the complexity of "y_." Examples might include using specific key performance indicators (KPIs) to gauge project success or leveraging longitudinal data to analyze trends in social behaviors.
- Data Collection and Reliability
The methods used for collecting data on "y_" directly influence the reliability of the measurements. Ensuring data quality involves careful consideration of sampling techniques, data integrity, and potential biases. Reliable data is essential for building accurate models and making valid inferences about "y_." Examples might include rigorous statistical sampling procedures, measures to control for bias in surveys, or employing standardized testing protocols for assessments.
- Statistical Analysis and Interpretation
Valid conclusions from "y_" measurements necessitate appropriate statistical analysis. Applying the correct statistical procedures to the gathered data is vital for extracting meaningful insights and avoiding misinterpretations. Appropriately chosen statistical methods can reveal trends, identify relationships, and quantify the significance of "y_." Examples include regression analysis to model relationships between variables, or hypothesis testing to determine if observed differences are statistically significant.
In summary, accurate measurement of "y_" hinges on meticulous attention to defining units, selecting appropriate metrics, ensuring data quality, and applying rigorous statistical methods. This multi-faceted approach guarantees a solid foundation for comprehending "y_" and its complex interplay with other factors. Thorough measurement is fundamental to informed decision-making and the effective utilization of knowledge derived from "y_."
5. Interpretation
Accurate interpretation of "y_" is essential for deriving meaningful insights and actionable knowledge. The process of interpretation involves deciphering the significance of "y_" within its specific context, recognizing patterns, and drawing conclusions. Effective interpretation is crucial for understanding "y_"s influence on other factors and its implications in various fields.
- Contextual Understanding
Interpretation hinges on a deep comprehension of the context surrounding "y_." Factors such as historical trends, geographical location, socio-cultural norms, and technological advancements all contribute to a nuanced understanding. A thorough contextual analysis ensures that interpretations are not superficial or misleading, recognizing the intricate relationships between "y_" and its environment. For instance, interpreting economic data requires understanding the political climate, technological innovations, and social structures influencing economic conditions. Ignoring these context-dependent factors can lead to flawed conclusions.
- Identifying Patterns and Trends
Interpreting "y_" often involves identifying patterns and trends within data or observations. Careful analysis allows for the recognition of recurring themes, progressions, or deviations. By identifying patterns, one can anticipate future developments, project potential outcomes, and draw inferences about causality. For example, consistent trends in consumer spending data can reveal shifts in purchasing behavior and trends that inform strategic marketing decisions.
- Assessing Causality and Correlation
Interpreting "y_" involves distinguishing between correlations and causations. While correlations can reveal associations, causations suggest underlying relationships. Misinterpreting a correlation as a causation can lead to inaccurate conclusions and misplaced actions. For example, observing a correlation between ice cream sales and crime rates does not imply that one causes the other; a third, underlying factor might be influencing both.
- Drawing Meaningful Conclusions
Interpretation culminates in drawing meaningful conclusions about the significance of "y_." These conclusions should be supported by evidence and logical reasoning, providing a clear understanding of the observed patterns and their implications. For example, interpreting market research data might lead to conclusions about consumer preferences, influencing product development or marketing strategies.
In conclusion, interpreting "y_" requires a multifaceted approach that encompasses contextual understanding, pattern identification, causal analysis, and drawing meaningful conclusions. By carefully considering these elements, interpretations become more insightful, allowing for a deeper understanding of "y_"s complex role and influence across various domains.
6. Relationship
Understanding the relationships of "y_" is critical. These relationships delineate how "y_" interacts with other variables, influencing its impact and interpretation. Examining these connections reveals the complexities of "y_" and its multifaceted influence. This exploration emphasizes the significance of these relationships for a comprehensive analysis of "y_."
- Interdependence with Other Variables
Relationships between "y_" and other variables often demonstrate interdependence. Changes in one variable frequently affect the other, creating dynamic interactions. For example, fluctuations in economic growth ("y_") can influence consumer confidence and investment decisions, illustrating a direct interdependence. Understanding these interdependencies is vital for forecasting future trends and anticipating potential outcomes.
- Causality and Correlation
Establishing the nature of the relationship causal or correlational is crucial. A causal relationship suggests one variable directly affects another. A correlational relationship indicates an association between variables, but not necessarily a direct cause-and-effect link. Determining the precise nature of this relationship enhances the accuracy and reliability of analysis and conclusions regarding "y_." For instance, identifying a correlation between education levels and income levels does not necessarily imply that increased education causes higher income; other factors might contribute.
- Magnitude and Direction of Influence
Understanding the magnitude and direction of influence is essential. The strength of the relationship between "y_" and other variables dictates its impact. A strong relationship suggests a substantial influence; a weak relationship signifies a limited impact. Likewise, the direction of the relationship (positive or negative) indicates the nature of the influence. For example, a positive relationship between advertising spending and sales indicates that increased advertising tends to correlate with increased sales.
- Mediating and Moderating Variables
Mediating and moderating variables can influence the relationship between "y_" and other factors. A mediating variable explains how one variable affects another, while a moderating variable alters the strength or direction of the relationship. Identifying and analyzing these variables provide a more comprehensive understanding of the intricate web of relationships surrounding "y_." For instance, a mediating variable might explain how "y_" (stress levels) influences physical health (outcome) through a mediating variable like sleep quality. Similarly, a moderating variable might modify the effect of stress levels ("y_") on physical health depending on the individual's coping mechanisms.
In conclusion, analyzing the relationships of "y_" to other variables is paramount for a complete understanding. By exploring interdependence, causality, magnitude, and moderating influences, one gains a deeper insight into the complex interplay shaping "y_" and its outcomes. This deeper understanding is critical for making accurate predictions, drawing reliable conclusions, and effectively applying knowledge about "y_" to diverse applications.
7. Impact
The impact of "y_" encompasses the consequences and effects stemming from its presence, actions, or influence. Understanding this impact is crucial for assessing the significance and implications of "y_" in various contexts. This analysis delves into key facets of the impact, emphasizing its role in shaping outcomes and driving change.
- Magnitude and Scope of Effects
The magnitude and scope of "y_"s impact are critical determinants in understanding its significance. A wide-reaching impact signifies extensive influence on various factors, whereas a narrow impact suggests limited influence. Factors like the scale of involvement, the number of affected entities, and the duration of impact determine the magnitude and scope. Examples include the global impact of climate change versus the localized impact of a specific policy. Evaluating the scope aids in prioritizing responses and allocating resources effectively.
- Short-term and Long-term Consequences
The impact of "y_" is often evaluated based on both its short-term and long-term consequences. Short-term impacts are immediate effects, while long-term impacts encompass consequences unfolding over extended periods. The evaluation should carefully consider the potential ripple effects over time. Recognizing the nuances between short-term and long-term implications is crucial for proactive planning and resource allocation. For instance, a company's decision to adopt a new technology might lead to immediate efficiency gains (short-term) but also potentially create new market opportunities or challenges down the road (long-term).
- Qualitative and Quantitative Assessment
Assessing the impact of "y_" often requires a multi-faceted approach incorporating both qualitative and quantitative methods. Qualitative measures explore the nature and characteristics of the impact, while quantitative measures quantify the magnitude and extent of the impact. Combining these methods provides a richer and more comprehensive understanding of the effects of "y_." For instance, evaluating the impact of a new educational program might include qualitative feedback from students and teachers and quantitative data on improved test scores. This integration offers a more balanced and meaningful evaluation.
- Intended and Unintended Consequences
Understanding the intended and unintended consequences of "y_" is essential for effective decision-making. Intended consequences are those anticipated results, while unintended consequences are unforeseen outcomes. Recognizing and analyzing these different types of consequences provides a complete picture of "y_"s impact and allows for proactive mitigation of potential negative outcomes. For example, a new law aimed at reducing pollution might have the unintended consequence of increasing energy costs for some industries.
In conclusion, evaluating the impact of "y_" necessitates a careful consideration of its magnitude, duration, qualitative and quantitative aspects, and both intended and unintended consequences. This multifaceted approach ensures a more holistic understanding, guiding effective planning, resource allocation, and decision-making in diverse contexts.
8. Comparison
Comparative analysis is indispensable for understanding "y_." By contrasting "y_" with other variables, factors, or situations, researchers can illuminate its unique characteristics, significance, and impact. Comparative studies reveal patterns, trends, and relationships, ultimately leading to a more profound comprehension of "y_." This process also helps identify potential causes and consequences, offering invaluable insights for decision-making and forecasting.
- Identifying Distinctions and Similarities
Comparing "y_" with alternative concepts, conditions, or scenarios highlights both its unique characteristics and shared attributes. By identifying distinctions and similarities, analysts can isolate the specific features of "y_" that drive its effects. For example, comparing the economic performance of different countries under various political systems can reveal factors influencing economic growth, such as regulatory frameworks, investment incentives, or workforce characteristics. Comparing success metrics across diverse industries illuminates common threads and industry-specific differentiators.
- Establishing Relative Value and Importance
Comparative analysis establishes a relative value scale for "y_." By measuring "y_" against relevant benchmarks, one can assess its significance in relation to other factors. For instance, comparing the efficiency of different production methods provides a framework for choosing the most cost-effective approach. Similarly, comparing the performance of different educational strategies allows for informed decisions regarding curriculum development.
- Unveiling Patterns and Trends
Comparing "y_" across various contexts, time periods, or populations often reveals underlying patterns and trends. By observing how "y_" behaves under different circumstances, one can deduce general principles or predict future behaviors. For example, comparing sales figures across different market segments can reveal purchasing patterns and preferences, useful for developing targeted marketing strategies. Studying demographic trends over time allows for forecasts regarding future populations and resource needs.
- Facilitating Predictive Modeling
Comparisons provide data for building predictive models. By analyzing patterns and similarities across various cases, researchers can develop predictive models incorporating "y_." These models can anticipate potential outcomes or project future trends. For example, historical stock market data can be compared with relevant economic indicators to construct predictive models that identify potential market fluctuations.
In conclusion, comparative analysis of "y_" provides a nuanced understanding of its characteristics and impact. Through comparisons, researchers can identify unique aspects, establish relative importance, uncover patterns, and build predictive models, ultimately maximizing the value derived from insights into "y_."
Frequently Asked Questions about "y_"
This section addresses common queries regarding "y_," providing concise and informative answers to promote a clearer understanding of its significance and applications.
Question 1: What is the precise definition of "y_"?
The precise definition of "y_" remains context-dependent. Without a specific context, a universally applicable definition proves elusive. The meaning of "y_" is best understood within the framework of the particular field or analysis in which it is employed. Operational definitions, which establish measurable parameters for assessing "y_," are essential for rigorous analysis.
Question 2: How is "y_" measured accurately?
Accurate measurement of "y_" hinges on carefully defining units, selecting appropriate metrics, and employing reliable data collection methods. The chosen metrics should align with the goals of the analysis. Valid conclusions depend on data quality and the application of rigorous statistical analysis techniques to ensure accuracy and avoid bias.
Question 3: What are the common misconceptions regarding "y_"?
A frequent misconception is equating correlation with causation. While correlations can reveal associations, they do not establish direct causal links. Furthermore, overlooking the contextual factors influencing "y_" can lead to misinterpretations of its significance and impact. An accurate understanding necessitates a nuanced perspective accounting for the surrounding circumstances.
Question 4: How does "y_" relate to other variables?
"y_" often exhibits complex relationships with other variables. These relationships can be interdependent, causal, or correlational. The magnitude and direction of these influences, as well as the potential mediating or moderating variables, must be carefully considered to avoid misinterpretations. Comprehensive analysis should explore these interdependencies to fully grasp "y_"s influence.
Question 5: What is the broader impact of "y_"?
The impact of "y_" is context-dependent and varies based on the specific application. Its significance extends to numerous fields, with various short-term and long-term consequences. Careful consideration of both intended and unintended outcomes is crucial for assessing the overall impact of "y_," particularly in complex systems and multifaceted scenarios.
In summary, "y_" requires careful examination and context-specific understanding. The provided responses emphasize the importance of precise definitions, appropriate measurement techniques, recognition of contextual influences, detailed analysis of relationships, and a comprehensive assessment of impact for a meaningful interpretation of "y_." The following section will delve into practical applications of "y_" in specific domains.
Next, we will explore specific use cases for "y_" in various sectors.
Conclusion
This exploration of "y_" underscores the multifaceted nature of the concept. Precise definition, accurate measurement, and a thorough understanding of context are essential for a meaningful interpretation. The intricate relationships between "y_" and other variables, coupled with the substantial impact it exerts, are key components for a complete analysis. The comparative analysis highlights the distinctive characteristics and relative significance of "y_" in various contexts. Furthermore, the nuanced evaluation of "y_"s impact, encompassing both intended and unintended consequences, is crucial for informed decision-making and effective problem-solving. The exploration demonstrates the necessity of a holistic approach acknowledging the interconnectedness of "y_" with its environment, be it social, historical, or technological.
Moving forward, a deeper understanding of "y_" necessitates continued investigation and rigorous analysis. The complexities inherent in "y_" demand a nuanced and multifaceted approach, acknowledging the intricate interplay of factors influencing its behavior and consequences. Future research should prioritize refining methodologies for measurement and interpretation, developing models that accurately predict the impact of "y_" across diverse settings, and fostering a collaborative environment for sharing knowledge and best practices related to "y_"s application.
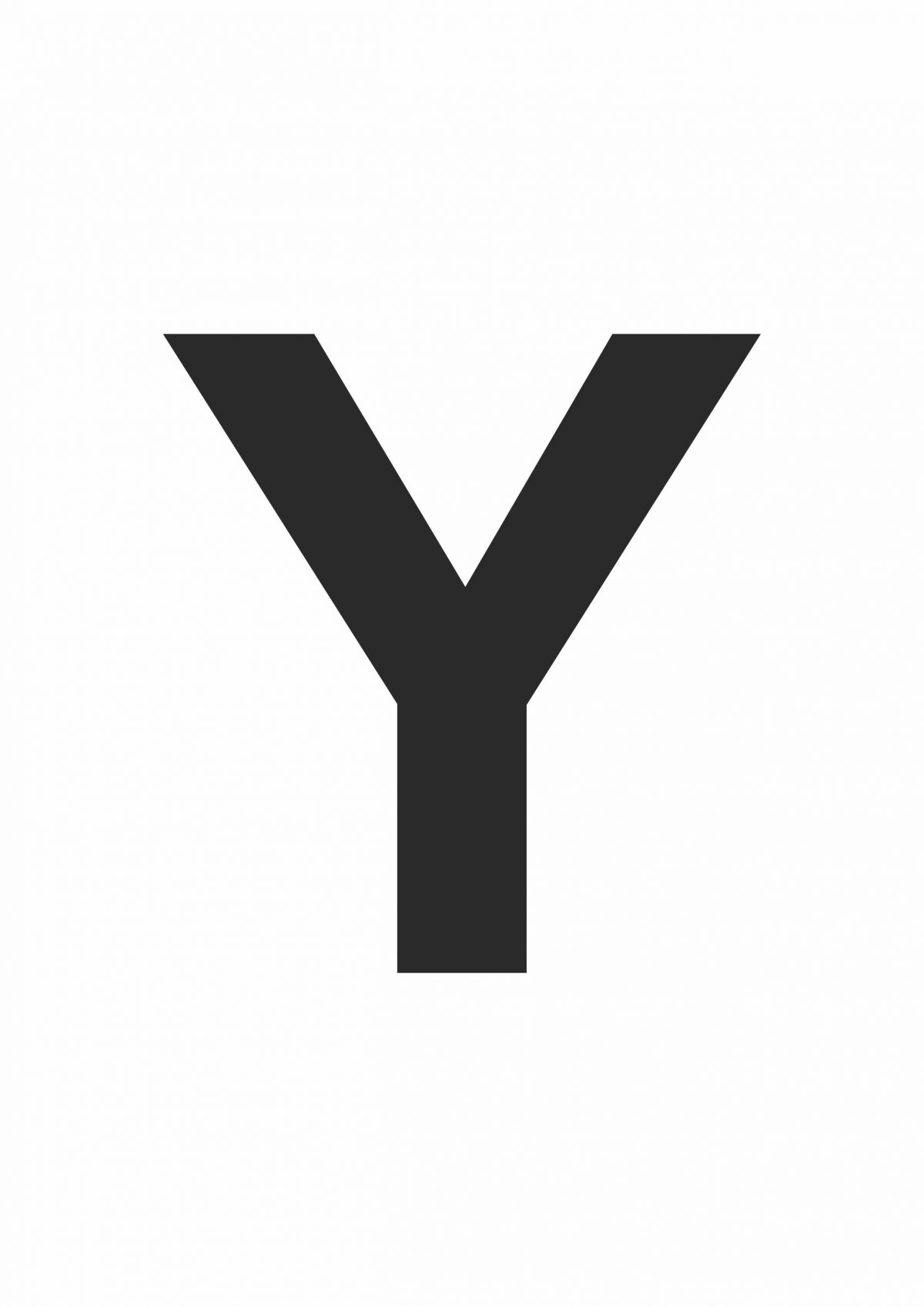
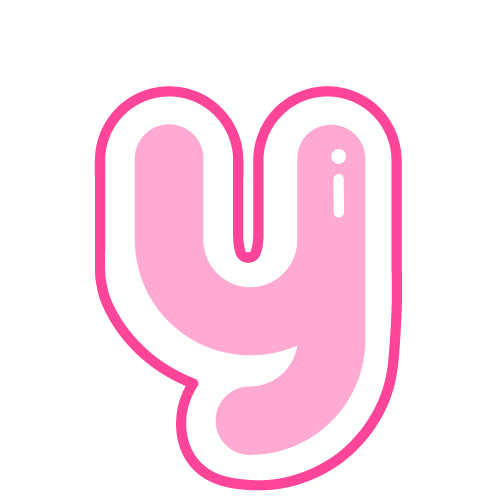
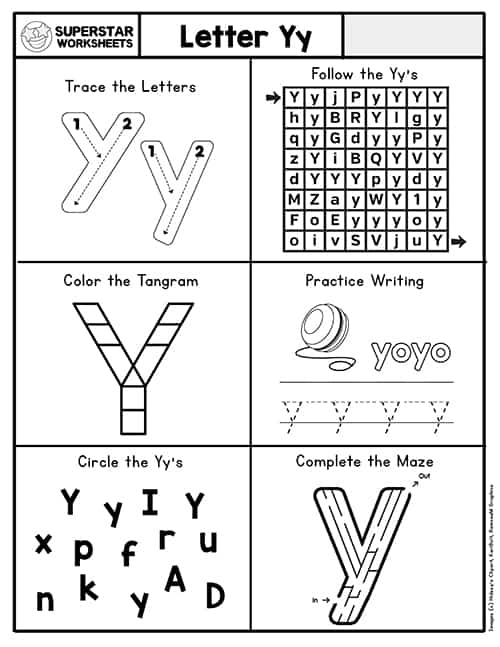