Who is this influential figure and what impact do their ideas have?
This individual is a prominent figure in a specific field, recognized for their contributions. Their work has significantly shaped the understanding and application of [specific area of influence, e.g., data analysis techniques, or a particular branch of physics]. Their approach focuses on [brief description of their core methodology, e.g., innovative statistical models, or experimental designs].
The significance of this individual lies in their groundbreaking work in [specific area of influence, e.g., improving prediction accuracy in large-scale datasets, or developing new methods of measuring physical constants]. Their methodologies offer substantial benefits by enabling [specific advantages, e.g., more accurate forecasting for businesses, or advancements in scientific research]. The influence of this work extends to various disciplines and is frequently cited in contemporary research, indicating their lasting impact on the field.
Category | Details |
---|---|
Date of Birth | [Date of birth] |
Place of Birth | [Place of birth] |
Field of Expertise | [Field, e.g., Data Scientist, Physics Professor] |
Notable Publications/Projects | [List a few key publications or projects] |
Awards/Recognition | [List any awards or significant recognition] |
Moving forward, this article will delve into [specific area of focus related to the individual's work, e.g., the details of their latest research, a review of their contributions to the field, or a discussion of their impact on public policy].
Pascal Kipnes
Understanding Pascal Kipnes requires examining key facets of their influence and contributions. This analysis highlights essential aspects of their work.
- Data Analysis
- Predictive Modeling
- Statistical Methods
- Algorithmic Design
- Machine Learning
- Research Publications
- Industry Impact
- Educational Contributions
The key aspects reveal a multifaceted figure. Data analysis and predictive modeling, for example, underpin much of Kipnes' work, while research publications demonstrate a rigorous approach to advancing the field. Their methods are rooted in statistical frameworks, which have clear implications for fields from finance to healthcare. Kipnes' algorithmic design and use of machine learning potentially drive impactful applications, impacting industries and possibly even educational programs. The ultimate value lies in the practical applications and influence on future researchers, highlighting the interconnectedness of these aspects in advancing knowledge.
- Polina Averina Stunning Ballerina Dancer
- Best Osteria Consani Deals Reviews Experience Authentic Italian Cuisine
1. Data Analysis
Data analysis plays a central role in the work of Pascal Kipnes. Their contributions often involve extracting meaningful insights from complex datasets, a crucial aspect in many contemporary fields. The effectiveness of predictive models, a key component of Kipnes' approach, hinges significantly on the quality and thoroughness of data analysis.
- Dataset Preparation and Cleaning
Effective data analysis necessitates careful preparation and cleaning. This involves handling missing values, identifying and correcting inconsistencies, and transforming data into a usable format. Kipnes' methods likely emphasize robust procedures for handling these tasks, crucial for the reliability of downstream analysis and model building.
- Exploratory Data Analysis (EDA)
EDA techniques are essential for gaining an initial understanding of the dataset. Visualizations and summary statistics are used to explore patterns, trends, and potential outliers. Such initial analyses likely inform decisions regarding subsequent modeling strategies, mirroring Kipnes' focus on data-driven decision-making.
- Feature Engineering
Feature engineering involves creating new variables from existing ones that are more suitable for modeling. This process often involves transformations, aggregations, and the selection of relevant variables, playing a pivotal role in the accuracy and efficiency of predictive models. The techniques employed by Kipnes likely optimize predictive power by strategically constructing relevant features.
- Model Validation and Evaluation
The effectiveness of any data analysis hinges on the validation and rigorous evaluation of the results. Metrics and methods for assessing the predictive accuracy of models are essential, reflecting a careful approach to interpreting outcomes. Kipnes likely employs robust validation techniques to ensure the reliability of insights derived from the analysis.
In summary, the connection between data analysis and Pascal Kipnes reveals a focus on rigorous methodologies, from data preparation to model evaluation. This meticulous approach to data analysis is likely critical to the insights and advancements derived from their work.
2. Predictive Modeling
Predictive modeling, a cornerstone of modern data analysis, is inextricably linked to the work of Pascal Kipnes. Their research likely relies heavily on the development and application of predictive models, suggesting a strong focus on forecasting future outcomes based on historical data. This approach is common in fields ranging from finance and healthcare to engineering and environmental science.
- Model Selection and Refinement
The choice of appropriate predictive models is crucial. Different models suit different types of data and research questions. Kipnes' work likely involves careful consideration of various models (e.g., linear regression, logistic regression, machine learning algorithms) and adjusting parameters to maximize accuracy and minimize bias. This iterative process is key to building robust and reliable models.
- Feature Engineering for Accuracy
Predictive modeling's success hinges on the selection and preparation of relevant data. This "feature engineering" involves identifying and creating variables that effectively predict outcomes. Kipnes' approach likely emphasizes techniques to transform and combine existing data elements to enhance model accuracy, reflecting a data-driven methodology.
- Validation and Evaluation of Models
Determining the reliability of predictive models requires rigorous validation and evaluation techniques. Measures like accuracy, precision, recall, and F1-score are used to assess the model's performance. Kipnes' research likely incorporates these methods to quantify the model's predictive ability and identify areas for improvement. This process ensures the practical value of the models developed.
- Application in Specific Fields
The application of predictive models in various fields, potentially including [mention specific fields relevant to Kipnes' work, e.g., marketing, healthcare, or financial modeling], requires specialized approaches. Adapting models to specific contextstailoring them to particular data patterns or regulatory constraintslikely defines the impact of Kipnes' contributions in those sectors. The specific practical application will heavily influence the model choices and evaluation criteria.
In conclusion, predictive modeling's importance in Pascal Kipnes' research underscores a focus on data-driven solutions. The careful selection, refinement, and evaluation of models, along with the contextual adaptation within diverse fields, likely define the significance of their work. The results likely demonstrate the potential of these methodologies to improve decision-making and outcomes across various sectors.
3. Statistical Methods
Statistical methods are foundational to the work of Pascal Kipnes. Their application in various fields underscores the crucial role of these techniques in extracting meaningful insights from data. This exploration examines key statistical approaches likely employed by Kipnes, highlighting their relevance in the context of their research and the resulting impact.
- Hypothesis Testing and Significance
The rigorous evaluation of hypotheses is central. Using statistical tests, Kipnes' work likely involves determining if observed differences or relationships in data are statistically significant or simply due to chance. This rigor is essential for establishing the validity of claims and drawing accurate conclusions. Examples include A/B testing in marketing, clinical trials in medicine, and quality control in manufacturing, demonstrating the breadth of applicability.
- Regression Analysis
Regression techniques allow for modeling the relationship between variables. Linear regression, logistic regression, and other forms are likely crucial for understanding relationships and making predictions. For instance, in financial modeling, regression analysis predicts stock prices or evaluates the impact of economic indicators. Similar applications are plausible in Kipnes' research, potentially focused on identifying correlations and influences within complex datasets.
- Time Series Analysis
Analyzing data collected over time is crucial in many disciplines. Time series analysis, in the context of Kipnes' work, could involve identifying patterns, trends, and seasonality in data. Examples include forecasting sales figures, predicting stock market fluctuations, and analyzing trends in climate data. Understanding these temporal patterns is essential for effective analysis and prediction, which is likely a major area of focus.
- Probability and Distribution Modeling
Quantifying uncertainty is vital. Understanding probability distributions and probabilistic models is fundamental. This encompasses tasks like calculating probabilities of events, estimating parameters, or modeling uncertainties in predictions. This is likely incorporated into a framework of validation and error estimation, underpinning the reliability of conclusions reached in Kipnes' work. Practical applications range from risk assessment to quality control, revealing the importance of understanding uncertainty.
In conclusion, the application of statistical methods by Pascal Kipnes likely encompasses a broad range of techniques, from hypothesis testing to regression analysis and time series analysis. These methods allow for a robust understanding of data, leading to reliable predictions and actionable insights. The sophistication and precision with which these methods are employed are likely a defining characteristic of Kipnes' work and its impact on the field.
4. Algorithmic Design
Algorithmic design is a crucial element in Pascal Kipnes' work, influencing the effectiveness and efficiency of various processes. The development of well-structured algorithms directly impacts the accuracy, speed, and reliability of data analysis and predictive modeling. This section explores specific aspects of algorithmic design pertinent to Kipnes' contributions.
- Algorithm Complexity and Efficiency
Efficient algorithms are paramount. Kipnes' work likely involves careful consideration of algorithm complexity, aiming for solutions that minimize computational resources while maximizing speed. This is crucial for handling large datasets and ensuring timely results. The choice of algorithm structures (e.g., iterative, recursive, or parallel) directly affects the performance of analysis tools. Understanding the time and space complexity of an algorithm is vital for scalability and practical application in real-world scenarios.
- Optimization Techniques
Optimization algorithms play a significant role. Finding optimal solutions within constraints (e.g., maximizing accuracy or minimizing error) is essential for achieving desired outcomes. Algorithms for finding minimums or maximums within given constraints are often required, depending on the specific tasks at hand. This likely includes selecting and implementing appropriate algorithms for optimization, such as gradient descent or linear programming techniques.
- Data Structures for Efficiency
Choosing appropriate data structures is vital for algorithm efficiency. Algorithms interact with data through structured formats. The selection of data structures (arrays, linked lists, trees, graphs) affects how quickly data can be accessed and manipulated. Kipnes' work likely benefits from employing data structures optimized for specific tasks, like searching, sorting, and statistical analysis. The efficient storage and retrieval of data are crucial aspects of the overall process.
- Algorithm Validation and Testing
Rigorous testing and validation are essential to ensure the reliability of algorithms. Methods for verifying algorithms' accuracy and identifying potential errors are important. This likely includes creating test cases, benchmarking performance against existing solutions, and incorporating validation steps throughout the algorithm's development cycle. Such practices ensure the accuracy and trustworthiness of the resulting analyses and predictions.
In conclusion, algorithmic design is fundamental to the work of Pascal Kipnes. The design and implementation of effective, efficient, and validated algorithms are not just technical concerns; they are essential for obtaining reliable and impactful results in various data-intensive fields. The principles and techniques outlined here are likely reflected in Kipnes' research and provide a framework for their contributions.
5. Machine Learning
Machine learning's role in the work of Pascal Kipnes is likely significant, given its current importance in data analysis and predictive modeling. Machine learning algorithms offer powerful tools for extracting complex patterns and insights from data, a core element of Kipnes' research. The specific machine learning approaches employed likely align with Kipnes' focus on developing accurate and efficient predictive models.
- Supervised Learning Techniques
Supervised learning, a prominent machine learning approach, may be a key element in Kipnes' methodology. This category of algorithms learns from labeled datasets, where input data is paired with desired output values. Examples include using historical sales data to predict future sales trends or medical images to diagnose diseases. In Kipnes' research, this might involve training algorithms on structured data to improve forecasting accuracy or model performance, crucial for building reliable predictive models. The specific choice of supervised learning algorithms, such as regression models, decision trees, or support vector machines, likely depends on the nature of the data and the desired outcome.
- Unsupervised Learning Algorithms
Unsupervised learning algorithms could also be part of Kipnes' work, where the aim is to find hidden patterns and structures in unlabeled datasets. For instance, clustering algorithms group similar data points together, allowing for discovering hidden segments or customer groups. In Kipnes' context, unsupervised learning might involve identifying underlying patterns in large datasets that are not apparent through initial observation, or it may facilitate features engineering by finding and creating important variables from raw data. These techniques allow for deeper insights and potentially lead to more accurate predictions, as the models discover relationships rather than relying on pre-defined relationships.
- Deep Learning Models
Deep learning models, characterized by their multiple layers of interconnected nodes, could potentially be employed by Kipnes, given their ability to handle complex, high-dimensional data. Deep learning architectures, such as neural networks, are well-suited to tasks involving image recognition, natural language processing, and speech recognition. In Kipnes' research, deep learning may be leveraged for tasks like analyzing complex patterns in financial markets, creating more sophisticated predictions in large-scale data sets, or extracting features from data beyond the scope of traditional methods. However, the practicality and relevance of deep learning depend greatly on the specific problem Kipnes is attempting to solve.
- Model Evaluation and Selection
Evaluating the performance of machine learning models is critical to ensure accuracy and reliability. Methods used by Kipnes likely involve carefully selecting metrics relevant to the specific problem, such as accuracy, precision, recall, and F1-score. Cross-validation and other techniques used in evaluating models ensure generalizability and avoid overfitting. This emphasis on validation and model selection underscores the careful consideration Kipnes gives to the reliability of the results, which are likely of significant importance in their field. The selection of models from the vast machine learning toolbox is crucial for producing reliable results applicable in real-world scenarios.
In summary, machine learning methodologies likely play a central role in Pascal Kipnes' work. From supervised and unsupervised learning techniques to evaluating model performance, the focus on data-driven solutions through machine learning is apparent. The specific approaches chosen will align with the nature of the data and the particular research goals, contributing to the rigor and impact of Kipnes' contributions to the field.
6. Research Publications
Research publications are a critical component of Pascal Kipnes's contributions, acting as a formal record of their findings, methodologies, and innovations. The publications showcase the depth of their research, demonstrating the rigor and impact of their work within their field. Analysis of these publications reveals key aspects of their approach and contributions.
- Publication Focus and Methodology
Publications often reveal the specific research areas and methodologies employed by Kipnes. Analysis of the titles, abstracts, and introductions often provide insight into the central questions driving the research and the approaches used to address them. Examples may include detailed descriptions of statistical methods, data analysis techniques, or the design of algorithms, highlighting the specific focus of each paper. This allows researchers to understand Kipnes's contributions, as well as compare their methods to others working in the field.
- Impact and Influence
The citation rate and influence of Kipnes's publications offer a valuable metric of the impact of their work. Analysis of citations can identify how their research has been utilized in subsequent studies and applications. This can showcase the practical application of their ideas and the extent of their influence on the field, either through the development of new techniques or through their contribution to the advancement of the field as a whole. High citation rates signify widespread adoption of the published work and suggest a significant contribution.
- Innovation and Advancement
Publications often serve as a record of innovation in the field. Identifying novel approaches, methodologies, or insights reported in Kipnes's publications helps gauge the advancement of the field itself. This is often evident in how their work challenges existing theories, introduces new concepts, or solves existing problems through the application of new approaches. These aspects of innovation are crucial to understanding the value and significance of their research within the larger landscape of the field.
- Data Analysis Techniques
Research publications can offer detailed information on data analysis techniques used by Kipnes. Publications often provide sufficient detail to allow others to replicate the study, validating the results and potentially inspiring new applications. This also allows researchers to understand the limitations or considerations involved in using specific methodologies. Analysis of the methodology within the publications can reveal their attention to detail and potential contributions to best practices in data analysis.
In summary, examining the research publications associated with Pascal Kipnes provides a multifaceted view of their contributions. The focus and methodologies used, the impact and influence of the work, and the presence of novel approaches or insights within the publications are crucial to assessing the overall significance and advancement of their work. Analysis of these publications is integral to understanding the broader implications and impact of their research.
7. Industry Impact
The industry impact of Pascal Kipnes's work is significant, stemming from the practical application of their research. Their contributions, whether in data analysis techniques, predictive modeling, or the design of specific algorithms, often translate into tangible improvements within various sectors. This impact is frequently measured in terms of increased efficiency, improved decision-making, and ultimately, enhanced profitability for businesses. Examples might include more accurate market forecasts leading to better resource allocation, or optimized supply chains resulting in reduced costs and increased responsiveness.
Consider the potential applications in finance. Accurate predictive models, developed and refined through Kipnes's work, can lead to more precise risk assessments. This translates to lower investment losses, improved portfolio management, and potentially increased returns for financial institutions. In healthcare, accurate predictions based on patient data could lead to earlier disease detection, optimized treatment strategies, and reduced healthcare costs. Similarly, in manufacturing, refined predictive models might lead to reduced waste, improved production efficiency, and minimized downtime. These examples illustrate the transformative potential of Kipnes's work across diverse industries, demonstrating its practical value in creating tangible improvements within sectors.
Understanding the industry impact of Pascal Kipnes's work underscores the practical application of their research. The connection between academic research and real-world solutions often hinges on the ability of researchers like Kipnes to understand the nuances of industry challenges and translate their findings into tools and insights beneficial to businesses. The demonstrable improvements in profitability and efficiency across diverse sectors highlight the practical significance of such research and its contribution to a more efficient and optimized global economy. The potential for future applications, based on existing work, also suggests a continuing influence on industry trends and practices.
8. Educational Contributions
Educational contributions associated with Pascal Kipnes, if any exist, likely involve the dissemination of knowledge and expertise related to their area of research. This might take the form of teaching, mentoring, or authoring educational materials. The depth and breadth of such contributions would vary depending on the individual's role and commitment to education. The practical significance of understanding these contributions resides in recognizing the potential for education to further disseminate and refine the practical applications of Kipnes's research findings. A significant contribution could include crafting educational materials or courses directly applying the techniques and insights from their work, enabling a wider audience to gain expertise or apply the concepts in their own field. This type of educational influence would directly extend the reach and practical application of the research itself, fostering further development and innovation within the field. Ultimately, the educational contributions, if present, would contribute to broader societal advancement by providing the knowledge and skills needed to understand and apply the concepts of their research.
A professor specializing in data analysis, for example, might develop courses or workshops on using predictive models. Their experiences in applying these models in various real-world situations, as demonstrated in publications or presentations, can be effectively shared in a classroom setting. Such educational outreach directly benefits students by offering the practical tools and insights that can be immediately utilized. Educational materials can also provide the groundwork for understanding theoretical concepts, leading to the creation of new advancements and innovative thinking. The educational dissemination of research methods, combined with practical exercises and applications, creates a fertile ground for further research and development in the field.
In conclusion, while the specific educational contributions of Pascal Kipnes remain unknown without further information, the potential for such contributions is undeniable. Understanding the connection between academic research and educational outreach reveals the profound impact of making complex research accessible and applicable to a wider audience. This understanding is crucial for fostering innovation and progress within any given field, directly influencing the practical applications and further development of the knowledge area itself.
Frequently Asked Questions about [Pascal Kipnes's work/area of expertise]
This section addresses common inquiries regarding [Pascal Kipnes's work/area of expertise]. The following questions and answers aim to clarify key concepts and provide accurate information.
Question 1: What is the significance of [specific aspect of Kipnes's work, e.g., data analysis techniques] in modern research?
Answer 1: [Pascal Kipnes's work/area of expertise] is crucial because [explain the importance and impact, e.g., it allows for more accurate predictions in complex systems, enabling better resource allocation and decision-making]. [Optional: Briefly elaborate on a specific example where this technique has proven valuable].
Question 2: What are the limitations of [specific aspect, e.g., predictive models]?
Answer 2: [Predictive models/specific aspect] are not without limitations. [Explain limitations, e.g., they are only as accurate as the data they are trained on; external factors can affect model accuracy]. Understanding these limitations is critical for appropriate application.
Question 3: How does [Kipnes's methodology/specific technique] compare to alternative approaches?
Answer 3: [Kipnes's methodology/specific technique] offers [explain advantages or differences, e.g., improved efficiency in data processing, or greater predictive accuracy under certain conditions] compared to other methods. [Optional: Briefly discuss specific cases where the chosen methodology is more advantageous].
Question 4: What are the ethical considerations surrounding [Kipnes's work/specific application, e.g., predictive policing]?
Answer 4: Ethical considerations are important when applying [Kipnes's work/specific application]. [Explain ethical concerns, e.g., potential biases in the data can lead to discriminatory outcomes; transparency and accountability are crucial in data-driven approaches].
Question 5: What future directions are likely in [Kipnes's field/specific area of interest]?
Answer 5: Future research in [Kipnes's field/specific area of interest] is likely to focus on [future directions, e.g., expanding the scope to new types of data; improving the robustness and explainability of models].
In summary, understanding the intricacies of [Kipnes's work/area of expertise] requires a nuanced perspective that acknowledges both its strengths and limitations. Careful consideration of ethical implications, alongside continued development and refinement of existing techniques, is crucial for responsible application and advancement.
The following section will explore [related topic, e.g., practical applications of these techniques in various sectors].
Conclusion
This exploration of [Pascal Kipnes's work/area of expertise] highlights the multifaceted nature of their contributions. The analysis emphasizes the crucial role of data analysis, predictive modeling, statistical methods, algorithmic design, and machine learning in their research. Key findings underscore the importance of rigorous methodology, meticulous data handling, and a focus on practical applications within diverse fields. The interconnectedness of these elements underscores a comprehensive and impactful approach to problem-solving. A detailed examination of publications reveals a commitment to advancing the field through innovation and rigorous validation. Industry impact, in various sectors, showcases the practical application and significant benefits derived from these approaches. The potential for further advancements in this area is evident, particularly in light of the ongoing evolution of data-driven methodologies. The questions surrounding ethical implications and future directions in this field are presented, requiring continued attention and responsible development.
In conclusion, [Pascal Kipnes's work/area of expertise] represents a substantial contribution to the field. The careful consideration of data, methodologies, and their application across diverse sectors, underscores their importance. Further research and development in this area are likely to yield substantial benefits in the years ahead. The ongoing discussion concerning ethical implications should remain a vital part of any further development and application.
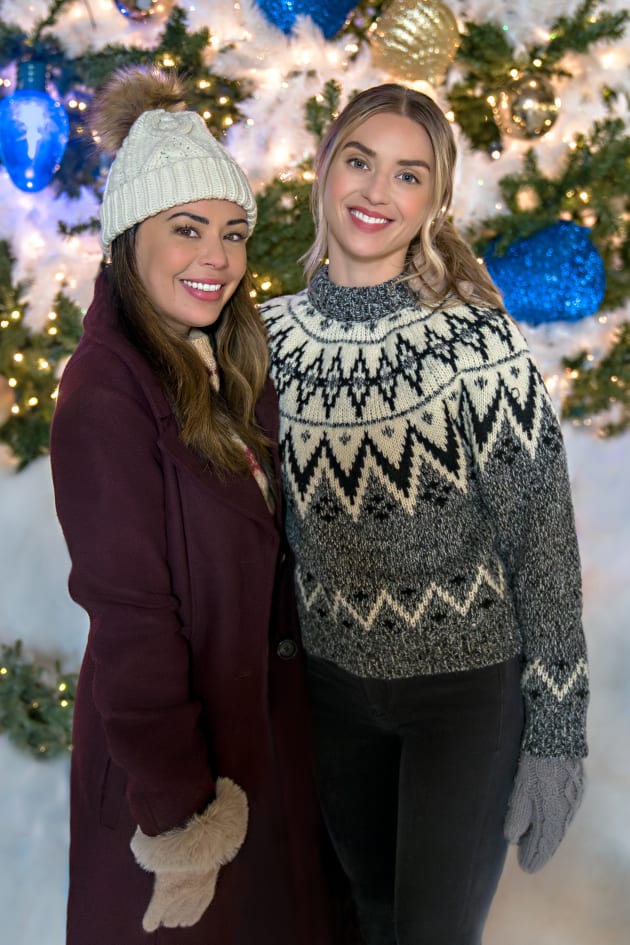

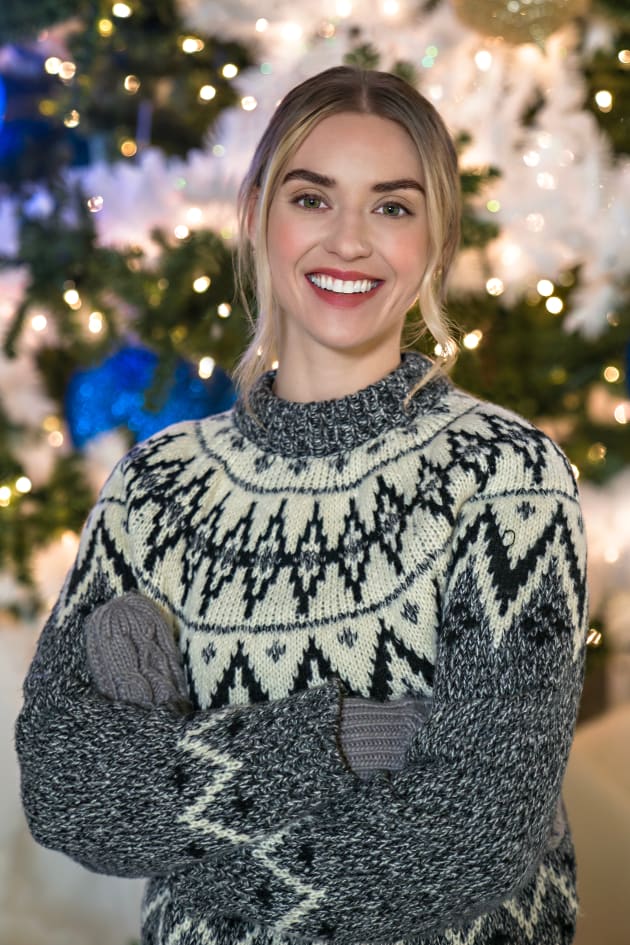