What is the significance of this sampling method? How does it ensure reliable data collection?
This sampling technique, a cornerstone of survey methodology, emphasizes the importance of representative samples in statistical studies. It prioritizes selecting participants from a larger population in a way that accurately reflects the characteristics of that population. For example, if a study aims to understand public opinion on a specific policy, a representative sample ensures the opinions gathered are not skewed by specific demographics being over-represented. The method often involves stratification and clustering to target specific groups within a population, thereby yielding more precise results. The core principle is to maintain the integrity of the data source and avoid bias.
The methodology's importance lies in its ability to produce reliable and generalizable results. It's foundational for drawing inferences about the wider population based on the study sample. This approach provides a robust framework for social research across diverse fields. The historical context of this method showcases its evolution and adaptation over time, from simple random sampling to more complex techniques. This evolution has improved the precision and accuracy of surveys, impacting public opinion research, market analysis, and academic studies alike.
Name | Role | Area of Expertise |
---|---|---|
(Name of Bert Kish, if known) | (Role or profession of Bert Kish, if known) | (Area of expertise or contribution, if known) |
Moving forward, we will delve deeper into the practical application of this methodology, highlighting various sampling designs and their potential pitfalls.
Bert Kish
The work of Bert Kish is crucial in the field of survey methodology. His contributions have shaped the understanding of sampling techniques and data collection.
- Sampling Methods
- Survey Design
- Data Analysis
- Non-response Bias
- Questionnaire Design
- Population Representation
Bert Kish's contributions are foundational to survey research. Sampling methods, crucial for representative data, are built upon his work. Expert survey design, encompassing all elements from questionnaire structure to population representation, benefits from his principles. His insights into non-response bias provide an important perspective for mitigating data distortion. Understanding how to accurately represent a population within a survey stems from principles he articulated. Thorough questionnaire design, ensuring accurate responses, is a key output of his influence. Improved data collection, and analysis, are all demonstrably related to the principles advocated by Kish. His work establishes clear guidelines for robust survey methodology, fostering accuracy and reliability in statistical research.
1. Sampling Methods
Sampling methods are fundamental to the work of Bert Kish. His extensive research emphasized the importance of selecting samples that accurately reflect the characteristics of a larger population. This concept is critical for producing reliable data in studies ranging from public opinion polls to market research. A poorly designed sampling methodology can lead to biased results, rendering conclusions inaccurate and potentially misleading. For instance, a poll seeking public sentiment on a new tax policy, if not sampling diverse demographics accurately, might not represent the full spectrum of opinions, therefore undermining the credibility of the findings.
Kish's work highlighted various sampling techniques, including stratified sampling and cluster sampling. These methods, often complex, are employed to ensure representation. Stratified sampling, for example, involves dividing a population into subgroups and then taking random samples from each stratum. This approach is particularly valuable when studying populations with significant differences within them. In contrast, cluster sampling is effective when the population is dispersed geographically or otherwise difficult to access. Kish's emphasis on these techniques allowed researchers to avoid biases arising from uneven representation, leading to more accurate estimations of population characteristics.
Understanding the connection between sampling methods and Kish's work has profound implications for research design. Accurate sampling ensures that research findings are generalizable to the broader population, increasing the validity of conclusions. Without sound sampling practices, drawing accurate conclusions from surveys and studies becomes precarious. This is important not only for academic research but also for policy decisions, business strategies, and a multitude of other applications requiring valid insights from populations.
2. Survey Design
Bert Kish's work profoundly influenced survey design principles. His emphasis on rigorous sampling techniques and data collection methods directly impacts the design of effective questionnaires and the overall structure of surveys. Survey design, in turn, relies on these principles to produce valid and reliable data reflecting the characteristics of a population.
- Question Formulation
Clear, concise, and unbiased question phrasing is paramount. Kish's insights highlight the potential for biased responses stemming from poorly worded questions. Examples include avoiding leading questions, ensuring questions are specific, and considering the respondent's understanding. This ensures the accuracy of data collected. Failure to adhere to these principles can skew results, leading to flawed conclusions and potentially misinformed decisions based on the survey.
- Response Options
Appropriate response options are critical to capture the nuances of respondent opinions or experiences. A limited set of options can restrict meaningful responses and limit the ability of the survey to gather valuable information. Kish's principles suggest designing options that accommodate diverse perspectives. This also includes considerations for scale type, avoiding ambiguity in response options and ensuring comprehensive coverage of possible answers.
- Questionnaire Structure
Logical flow and organization within the questionnaire are vital for respondent engagement and accurate data collection. Kish's approach to survey design emphasizes a clear order and structure, ensuring questions are logically sequenced. This aspect of design aims to minimize respondent fatigue and improve the accuracy and completeness of the responses. A poorly structured questionnaire can lead to respondent confusion and incomplete data sets.
- Pilot Testing
Rigorous pre-testing of questionnaires is crucial to identify potential issues and refine the survey's effectiveness before wider implementation. Kish's emphasis on sampling accuracy extends to the pre-testing phase. This involves identifying any ambiguities, inconsistencies, or respondent comprehension problems in the wording, format, or organization of questions, allowing adjustments before the formal survey is conducted.
These facets of survey design, directly informed by Kish's principles, are essential for obtaining valid and reliable data. Sound survey design, built on these components, enhances the integrity and usefulness of the data collected, directly contributing to the value of any research or study relying on survey methodology.
3. Data Analysis
Data analysis, a critical component of statistical research, is inextricably linked to the work of Bert Kish. Kish's emphasis on sound sampling methods directly influences how data collected through surveys is analyzed. Accurate representation of a population within a sample dataset is fundamental to valid inferences. Analysis of data, therefore, hinges on the integrity and appropriateness of the sampling strategy employed.
- Descriptive Statistics
Descriptive statistics, summarizing and presenting data characteristics, are fundamental in any analysis. Kish's principles ensure the data accurately reflect the population from which the sample was drawn. For example, analyzing the mean income in a survey depends on the representativeness of the sample for the broader population of interest. Appropriate sample selection minimizes distortions arising from skewed representation.
- Inferential Statistics
Inferential statistics allow researchers to draw conclusions about a population based on sample data. This process relies on sound statistical models, and the accuracy of these models, in turn, depends on the appropriateness of the sampling frame. If the sampling method deviates from Kish's principles, the confidence in the inferential conclusions diminishes, potentially leading to misleading estimations. For instance, an analysis of voter preference based on a sample that doesn't represent demographic diversity will not offer reliable projections for the entire electorate.
- Bias Detection and Mitigation
Kish's work emphasizes the identification and control of biases in data. Analysis necessitates examining potential biases introduced during data collection. Identifying non-response bias, coverage bias, or interviewer bias and correcting for them, where possible, are crucial steps informed by Kish's principles. Strategies for mitigating these biases, tailored to specific situations, enhance the validity of the research.
- Sampling Error and Precision
Sampling error, inherent in any sample-based study, is a key consideration. Kish's contributions provide frameworks for understanding and quantifying this error. Analysis should account for this inherent uncertainty. The size and variability of the sampling frame, together with appropriate sampling methods, dictate the precision of estimations, which is a direct result of the sampling methodology informed by Kish's work. Understanding sampling error allows researchers to create accurate confidence intervals around estimations.
In conclusion, the principles championed by Bert Kish are crucial to robust data analysis. From understanding the importance of sampling method to examining potential bias and calculating sampling error, these principles form the backbone of sound statistical practice. Data analysis methods employed must be contextually suited to the chosen sampling strategy to avoid misinterpretations and ensure reliable insights.
4. Non-response Bias
Non-response bias is a critical concern in survey research, directly impacting the validity and reliability of data. Bert Kish's work emphasized the importance of representative samples, and non-response bias significantly undermines that representation. Understanding this bias is fundamental to accurately interpreting results and acknowledging limitations in research employing survey methods.
- Definition and Types of Non-response Bias
Non-response bias arises when individuals selected for a sample choose not to participate in the survey. This creates a difference between the characteristics of the sampled population and the characteristics of the individuals who actually responded. Various forms of non-response bias exist. For instance, individuals with strong opinions, regardless of the subject, may be more likely to participate. Conversely, those with less interest or potentially those who oppose the research topic may not participate, skewing results. This selective participation can affect conclusions drawn about the entire population.
- Impact on Generalizability
The impact of non-response bias is that the study sample may not accurately reflect the characteristics of the larger population. Consequently, findings might not be generalizable to the wider population, thereby diminishing the validity of the conclusions drawn. For example, a survey regarding public support for a tax increase, if disproportionately completed by those who strongly favor or oppose the tax, will provide an incomplete or biased picture of the overall sentiment.
- Methods for Minimizing Non-response Bias
Kish's emphasis on sampling techniques, along with survey design, often directly informs methods for minimizing non-response bias. Strategies may include incentives for participation or modifications to the survey design to improve clarity or respondent engagement. Follow-up efforts and diverse strategies for contacting individuals are also necessary to reduce bias from non-respondents. This aims to reduce the gap between the sampled population and the responding group, bolstering the validity of conclusions about the broader group.
- Connection to Sampling Frameworks
The selection of sampling methods, as emphasized by Kish, is directly relevant to the management of non-response bias. Careful planning and the use of strategies like stratified or clustered sampling can help target specific groups within the population. These approaches can help account for non-response patterns across various strata, thereby reducing bias and improving data reliability.
Non-response bias is a pervasive issue in survey research, undermining the goal of representative sampling. Strategies employed to address this bias, often informed by Kish's work, are necessary to mitigate its effect and produce more reliable and generalizable findings. Addressing this bias directly enhances the validity and credibility of survey-based conclusions, making the research more trustworthy and impactful.
5. Questionnaire Design
Questionnaire design is intrinsically linked to the principles of sampling and survey methodology championed by Bert Kish. A well-designed questionnaire is crucial for accurate data collection, ensuring the reliability and validity of results. Kish's emphasis on representative samples necessitates that the questions employed in a survey accurately capture the views and experiences of the target population. Inadequate questionnaire design can lead to biased responses, invalidating findings. For example, leading questions or ambiguous wording can sway respondents toward particular answers, undermining the integrity of the entire research process. Conversely, a skillfully crafted questionnaire, built upon the principles of clarity, neutrality, and comprehensiveness, improves data quality and allows researchers to draw accurate conclusions from the collected data.
Several key aspects of questionnaire design are directly influenced by Kish's work. First, the clarity and conciseness of questions are paramount. Ambiguous or overly complex questions can lead to misinterpretations, resulting in inaccurate data. Second, question wording must be free of bias. Leading questions, loaded language, or questions that imply a particular response can skew results, obscuring the true sentiment of the respondents. Third, the structure of the questionnaire matters significantly. A logical flow, clear groupings of questions, and an overall user-friendly design enhance respondent engagement and reduce the likelihood of errors or omissions. Consider, for instance, a survey designed to understand public opinion on environmental policies. Clear and concise questions about specific policies, phrased neutrally, and presented in a logical order significantly increase the likelihood of collecting accurate data, whereas poorly worded, biased, or confusing questions would undermine the survey's integrity. This impacts the reliability and credibility of any conclusions derived from the data.
In summary, effective questionnaire design is inextricably bound to the principles of sampling emphasized by Bert Kish. A well-structured questionnaire, aligned with these principles, ensures the collection of reliable data. Clear, concise, unbiased questions, logical flow, and careful pilot testing directly contribute to the validity and representativeness of the sample. By adhering to these principles, researchers ensure that data gathered accurately reflects the characteristics of the larger population. Any deviation from these principles can lead to skewed results, invalidating the entire research endeavor and diminishing the value of the research conclusions. This demonstrates the crucial importance of questionnaire design in survey research, fundamentally linked to achieving the goals of accurate, representative, and reliable findings.
6. Population Representation
Accurate population representation is a cornerstone of sound survey methodology, directly tied to the work of Bert Kish. Kish's contributions highlight the imperative of selecting samples that mirror the characteristics of the larger population being studied. This principle underpins the validity of any inferences drawn from survey data. A sample that fails to reflect the population's diversity introduces bias, leading to inaccurate conclusions. For example, a survey regarding public support for a new environmental policy, if it disproportionately samples residents of affluent neighborhoods, may produce results that overestimate support among the general population, as the affluent group may hold differing views compared to the broader community. Representativeness, therefore, is essential for drawing valid conclusions about the population's views and behaviors.
The concept of population representation is not merely theoretical. It has profound practical implications. In market research, a poorly representative sample can lead to inaccurate projections of consumer demand, causing companies to launch products or services that fail to gain traction among the target audience. In public health studies, samples that don't account for demographic variations can produce skewed results, potentially delaying the development of effective interventions for specific subgroups. Political polling, reliant on accurate population representation, can also be significantly affected by the lack of such considerations, potentially leading to an inaccurate understanding of voter intentions. These practical examples underscore the importance of meticulously designed sampling strategies to ensure accurate representation of the entire population. The practical significance of this understanding can impact everything from product development to public policy decisions.
In essence, accurate population representation is not an optional feature of survey research but a foundational requirement. Kish's work emphasizes the methodological steps crucial for achieving this. By carefully considering the diverse elements of the population and employing suitable sampling techniques, researchers can increase the likelihood of obtaining results that accurately reflect the overall characteristics and opinions of the entire population. This rigorous methodology provides the basis for reliable and valid inferences in social, political, and economic analyses. The challenges lie in identifying and accounting for potential biases that arise from limitations in sampling methods. Understanding these challenges within the context of Kish's work is key to responsible and meaningful research outcomes.
Frequently Asked Questions about Sampling Techniques
This section addresses common inquiries related to sampling techniques, particularly those influenced by the work of Bert Kish. These questions explore fundamental concepts and best practices for ensuring reliable and representative data in various research contexts.
Question 1: What is the significance of proper sampling in research?
Proper sampling is fundamental to research validity. A representative sample accurately reflects the characteristics of a larger population. This allows researchers to draw reliable conclusions about that population based on the smaller, studied sample. Without proper sampling, generalizations to the larger population become questionable and potential biases could significantly distort outcomes. For example, a survey of customer satisfaction regarding a new product, using a sample that predominantly includes long-time customers, may not accurately reflect the overall customer base and lead to faulty recommendations for product improvements.
Question 2: How does sampling minimize bias?
Sampling techniques, such as stratified or cluster sampling, aim to minimize bias by ensuring that the sample's composition mirrors the population's characteristics. This is achieved by strategically selecting participants across various subgroups. For instance, if surveying voter preferences, a sample stratified by age and geographical location could more accurately represent the electorate than a simple random sample. Proper stratification helps control biases associated with specific demographics.
Question 3: What is non-response bias, and how is it addressed?
Non-response bias occurs when selected participants do not respond to the survey. This can create a difference between the characteristics of the sampled population and those who actually respond, potentially skewing the results. Strategies such as incentives for participation, multiple contact attempts, and employing follow-up methods help to mitigate non-response bias and enhance the representativeness of the response group. For example, employing varied contact methods (phone, email, mail) and adjusting the survey process to reduce respondent burden helps address this issue.
Question 4: What role does questionnaire design play in sampling?
Questionnaire design plays a crucial role in sampling methodology. Clear, concise, and unbiased questions are essential. Leading or overly complex questions can skew responses. The design should minimize respondent burden and ensure a logical flow, improving the completeness and accuracy of responses. For example, a questionnaire focused on consumer product preferences should use neutral wording to avoid influencing consumer opinions and provide diverse response options relevant to the topic. Well-designed questionnaires increase the quality of the collected data.
Question 5: How does sampling error impact the findings of a study?
Sampling error is the difference between the characteristics of a sample and those of the larger population it represents. This is an inherent aspect of sampling, as a sample can never perfectly reflect every characteristic of the wider population. Understanding and quantifying sampling error is essential for interpreting survey results. Larger samples generally reduce sampling error and increase confidence in the generalizability of findings. Properly understanding sampling error enables researchers to establish confidence intervals around estimations and acknowledge the inherent uncertainty associated with sample-based research.
A thorough understanding of sampling techniques, informed by principles such as those championed by Bert Kish, is vital for producing valid and reliable research outcomes. The effective use of various sampling methodologies directly influences the ability of researchers to draw accurate conclusions from their studies.
Moving forward, we will explore specific sampling techniques and their applications in various fields of research.
Conclusion
Bert Kish's contributions to survey methodology are substantial and enduring. This exploration has highlighted the foundational role of sampling techniques in ensuring the validity and reliability of survey-based research. Key aspects examined include the importance of representative sampling, the complexities of non-response bias, and the meticulous attention required in questionnaire design. The principles emphasized by Kish, such as careful consideration of population representation and the potential for bias in data collection, are crucial for producing accurate and generalizable findings across various disciplines. The practical implications of applying these principles are evident in the necessity of representative samples for robust conclusions in market research, public opinion polling, and numerous other applications. Kish's work continues to shape current approaches to survey design, highlighting the enduring need for rigorous methodological practices.
The principles underlying sound survey methodology, directly informed by Kish's contributions, remain essential in the context of contemporary research. Researchers must continually strive for accuracy and reliability in sampling and data collection. The commitment to these principles, coupled with innovative methodologies and a critical evaluation of potential biases, assures robust and valuable insights. Furthermore, the ongoing evolution of survey techniques demands continuous refinement and adaptation based on emerging challenges and advancements in research methods. The legacy of Bert Kish provides a vital framework for maintaining high standards of integrity and validity in statistical research.


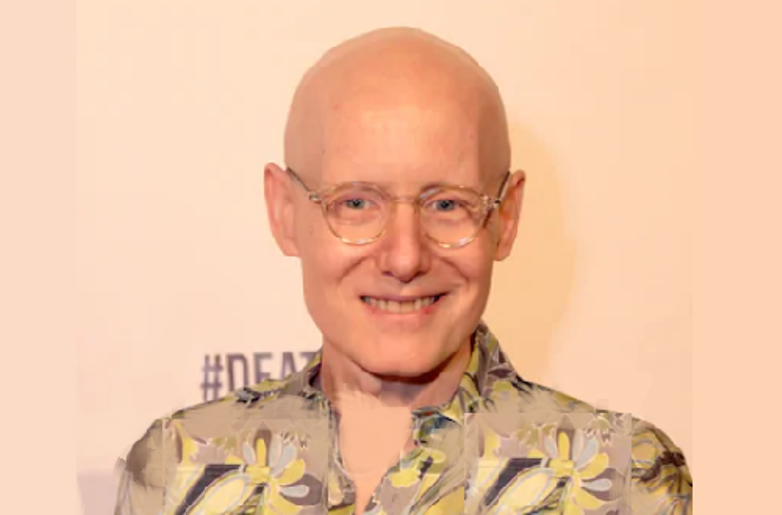