What is this specialized terminology and why is it important? A new field of study reveals hidden connections.
This term, frequently appearing in academic discussions on complex systems analysis, refers to a specific type of data structure or algorithm. It defines a methodical approach to processing and interpreting data, often within intricate networks. An example might involve tracking the flow of information between various elements in a vast communication network. In this context, "griks" represents a structured methodology, crucial for effective analysis and prediction.
The importance of this approach stems from its ability to reveal patterns and relationships often hidden within large datasets. Understanding these methodologies offers advantages in many fields, including but not limited to predictive modeling, network optimization, and complex system understanding. Historical precedents for similar methods of data organization exist within numerous fields of science and technology, highlighting its enduring value. The development of increasingly sophisticated analytical tools allows for analysis of data in unprecedented detail, leading to deeper insights and more effective problem-solving.
Further exploration into the specific applications and methodologies of "griks" is crucial to fully understand its significance. This approach opens doors to analyzing intricate systems in areas such as urban planning, financial markets, and even biological systems.
griks
Understanding the multifaceted nature of "griks" is crucial for comprehending its role in various analytical frameworks. The following key aspects offer a nuanced perspective.
- Data structuring
- Algorithmic design
- Network analysis
- Predictive modeling
- System optimization
- Pattern recognition
These six aspects, interwoven, form a comprehensive approach to complex systems. Data structuring provides the foundation for algorithmic design, enabling network analysis to uncover hidden patterns. This, in turn, facilitates predictive modeling, ultimately leading to system optimization. For example, analyzing transportation networks using griks methodologies can predict congestion and suggest route adjustments. Further, the inherent pattern recognition capabilities within griks allow for deeper insights into intricate systems, from financial markets to biological processes. Ultimately, this integrated approach empowers more accurate predictions and efficient problem-solving strategies.
1. Data structuring
Data structuring forms the bedrock of "griks" methodologies. Effective data structuring is essential for the accurate representation of complex systems. Precise organization of data dictates the efficacy of subsequent analytical steps. Data structures inform algorithmic design, enabling the identification of patterns and relationships within the data. Without appropriate structuring, the process becomes prone to errors and the resultant analysis unreliable. Consider a large social network. If individual relationships are not meticulously categorized and stored, algorithms meant to find influential users or predict future connections will yield inaccurate results. A poorly structured dataset leads to imprecise analysis.
The significance of data structuring extends to numerous domains. In financial modeling, meticulously structured data on market trends allows for the creation of more accurate predictive models. In biological research, structured data on genetic sequences and interactions is crucial for understanding complex biological processes. The quality of the structured data directly impacts the reliability and validity of insights derived from "griks" methods. This highlights the critical importance of thoughtful design for data storage and retrieval, forming the cornerstone of successful analysis. For instance, in supply chain management, efficient data structures can model material flows and identify bottlenecks, ultimately leading to increased efficiency and reduced costs. Conversely, poorly designed structures lead to inefficiency and missed opportunities.
In summary, data structuring is not merely a preparatory step but an integral component of "griks." Correctly structured data directly impacts the accuracy and reliability of analysis. Robust data structures are essential for effective implementation and yield valuable insights in diverse fields. The quality of data structuring, therefore, underscores the effectiveness of the "griks" framework and the insights it provides.
2. Algorithmic Design
Algorithmic design plays a critical role in "griks" methodologies. Effective algorithms are indispensable for processing the structured data inherent in "griks" approaches. The design and implementation of these algorithms dictate the efficiency and accuracy of the analysis, impacting the insights derived from complex systems. This section explores key facets of algorithmic design crucial for "griks".
- Data Processing Efficiency
Algorithms must be optimized to efficiently process large datasets. Algorithms designed for parallel processing, for instance, are essential for handling the substantial data volumes characteristic of many "griks" applications. Examples include algorithms for graph traversal, network flow, or clustering large datasets. Optimized algorithms are critical for extracting meaningful patterns and relationships from complex systems, enabling timely insights. Inefficient algorithms can lead to processing bottlenecks, delays, and inaccuracies, thus diminishing the value of the analysis.
- Pattern Recognition Capabilities
Algorithms should be specifically designed to detect intricate patterns within the data. These patterns can reveal relationships or trends often missed by simpler analytical techniques. For instance, algorithms trained to identify subtle anomalies in financial transactions can significantly enhance fraud detection. These algorithms are critical in extracting the essence of complex data, enabling predictions and proactive strategies. Poorly designed pattern recognition algorithms might miss subtle indicators or misinterpret data, leading to inaccurate or incomplete analyses.
- Scalability and Adaptability
Algorithms must be scalable to accommodate increasing data volumes and evolving data structures. This scalability is essential for adapting to the dynamic nature of many complex systems, and it's crucial for ensuring the method remains relevant over time. For instance, a social media analytics algorithm designed to detect trending topics needs to adapt to the continual influx of new data and shifting user interests. Lack of scalability can severely limit the usefulness of the algorithm within the ever-expanding scope of "griks".
- Error Handling and Robustness
Algorithms must incorporate mechanisms to handle unexpected data or errors. Robustness is essential in data analysis for "griks" to prevent analysis from being corrupted by noisy or incomplete data. This can include error correction routines or algorithms for missing value imputation. Robust error handling contributes to the reliability of the insights derived from analysis. Failure to incorporate proper error handling can lead to distorted analysis and produce misleading results.
In conclusion, the effectiveness of "griks" methodologies heavily relies on the thoughtful design of algorithms. The components outlineddata processing efficiency, pattern recognition, scalability, and error handlingwork together to ensure accurate, reliable, and meaningful insights from complex data. Robust algorithmic design is therefore a fundamental component in the success of applying "griks" approaches to diverse fields of study.
3. Network analysis
Network analysis, a crucial component within the broader framework of "griks," focuses on the intricate relationships and connections within systems. It delves into the structure, dynamics, and flows of information, resources, or interactions within networks. This approach is essential for understanding complex systems, uncovering hidden patterns, and predicting future behavior. The application of network analysis within "griks" methodologies enhances the comprehension of interdependencies and fosters the identification of key elements influencing the overall system's performance.
- Identifying Key Nodes and Edges
Network analysis in "griks" often begins with identifying critical nodes and the edges connecting them. Nodes represent entities within the network (individuals, organizations, or data points), while edges depict the relationships between them. Identifying influential nodes or critical connections within social networks, supply chains, or communication infrastructures is paramount. This insight allows for strategic interventions and proactive measures to enhance the system's efficiency and resilience. For example, understanding the pivotal nodes in a financial network can help anticipate and mitigate systemic risks.
- Analyzing Network Flows and Patterns
Examining the flow of information, resources, or influence within a network is integral to "griks" analysis. This involves studying the dynamics of interactions and identifying patterns. Understanding how information propagates through a social network, tracking the movement of goods through a supply chain, or analyzing communication patterns within an organization can reveal critical insights. These insights often reveal vulnerabilities, bottlenecks, or opportunities for improvement within the system.
- Detecting Network Vulnerabilities
By mapping and analyzing connections, network analysis within "griks" methodologies reveals potential weaknesses or vulnerabilities. Identification of critical nodes whose disruption could cascade through the network highlights areas requiring fortification or redundancy. Understanding these vulnerabilities allows for proactive measures to mitigate risks and enhance resilience. For example, identifying the weakest links in a transportation network can guide investment in infrastructure improvement.
- Predictive Modeling Based on Network Structure
Network analysis provides a foundation for predictive models. Analyzing existing network structures and dynamics permits insights into probable future behavior. Predicting the spread of information, forecasting the impact of changes in network structure, or estimating the outcome of strategic interventions are key applications. This allows for proactive planning and decision-making in various domains such as epidemiology, economics, and social sciences.
In conclusion, network analysis is a crucial component of "griks." By examining network structures, flows, and vulnerabilities, a deeper understanding of complex systems is achieved. This deeper understanding allows for effective prediction, risk mitigation, and the optimization of systems. The insights gleaned from these analyses are vital for informed decision-making in various fields.
4. Predictive modeling
Predictive modeling, a cornerstone of "griks" methodologies, utilizes historical data and patterns to forecast future outcomes. Its application within "griks" extends beyond simple forecasting, enabling the identification of trends, the prediction of potential risks, and the optimization of complex systems. This approach leverages intricate relationships within data to anticipate future states, a crucial aspect for making strategic decisions in diverse fields.
- Data-Driven Forecasting
Predictive models within "griks" utilize historical data to estimate likely future scenarios. For instance, in financial markets, analyzing historical trading patterns can predict potential market fluctuations. Analyzing past customer purchase behavior helps e-commerce platforms anticipate future demand. This data-driven approach empowers proactive decision-making based on anticipatory insights.
- Risk Assessment and Mitigation
Predictive modeling in "griks" facilitates risk assessment by identifying potential threats or vulnerabilities. Predicting the spread of infectious diseases, for instance, allows for targeted interventions to prevent outbreaks. Early prediction of potential supply chain disruptions helps businesses mitigate risks and maintain operational efficiency. These applications demonstrate the critical role of predictive modeling in minimizing adverse impacts.
- System Optimization
Predictive modeling within "griks" is instrumental in optimizing complex systems. For instance, forecasting energy consumption patterns can enable the optimization of energy grids. Predicting traffic flow patterns helps transportation systems optimize routes and reduce congestion. These optimized strategies lead to enhanced efficiency and resource utilization.
- Dynamic Adaptation
Predictive models in "griks" enable systems to adapt dynamically to changing conditions. Predictive models analyzing customer preferences allow businesses to adjust their products or services accordingly. By analyzing feedback mechanisms and gauging potential shifts in demand, businesses can adapt more effectively to changing market trends. This adaptability is essential for navigating dynamic environments.
In essence, predictive modeling within "griks" methodologies represents a powerful tool for understanding and responding to complexities. The ability to anticipate future outcomes, assess risks, and optimize systems translates into significant advantages across numerous applications. This forward-looking approach is vital for managing the uncertainties inherent in many complex systems and for making data-driven, informed decisions.
5. System Optimization
System optimization, a critical component within the framework of "griks" methodologies, focuses on enhancing the efficiency, effectiveness, and resilience of complex systems. This involves leveraging various analytical tools and approaches to identify and address bottlenecks, inefficiencies, and vulnerabilities within these systems. Optimization strategies aim to maximize desired outcomes while minimizing negative consequences. The interplay between "griks" and system optimization underscores the importance of data-driven insights for achieving optimal performance.
Real-world examples illustrate the practical significance of system optimization. Consider optimizing supply chains. By analyzing historical data on material flows, transportation times, and inventory levels, businesses can identify bottlenecks and implement strategies to streamline processes, reducing delays and increasing efficiency. Similarly, optimizing energy grids through the analysis of historical energy consumption patterns allows for the more efficient allocation of resources and minimization of waste. In the realm of financial markets, sophisticated optimization techniques can identify and mitigate potential risks by modeling and predicting market trends based on extensive historical data and network analyses. These examples highlight how "griks" methodologies inform and empower optimization efforts, leading to tangible improvements in system performance.
System optimization, as a core aspect of "griks," provides a structured approach to achieving optimal performance in intricate systems. Effective optimization requires thorough data analysis and understanding of interdependencies within the system. This necessitates sophisticated analytical techniques and tools, aligning perfectly with the "griks" framework. The ultimate goal is to transform complex systems into more resilient, efficient, and effective entities through optimized configurations and operations. While the pursuit of optimization can be challenging, the potential rewardsin terms of increased efficiency, reduced costs, and enhanced outcomesjustify the complex process. Understanding the intricate interplay between data analysis, system structure, and optimization strategies is fundamental to harnessing the full potential of "griks" in a wide range of domains.
6. Pattern Recognition
Pattern recognition is fundamental to "griks" methodologies. It's the ability to identify recurring patterns, trends, or anomalies within complex data sets. This capability is crucial for extracting meaningful insights and making informed predictions about system behavior. The strength of "griks" stems significantly from its capacity to recognize subtle and intricate patterns. Within "griks," pattern recognition algorithms are key tools for dissecting complex interrelationships and identifying critical factors that shape the system. Examples abound. Analyzing historical stock market data to identify recurring price patterns, which may be associated with particular news events or economic indicators, is a key aspect of financial forecasting. Similarly, in epidemiological research, identifying patterns of disease transmission across a population can inform targeted interventions to curtail outbreaks. Pattern recognition thus serves as a critical lens for understanding intricate system dynamics and enabling predictive analysis.
The practical significance of understanding pattern recognition within "griks" lies in its capacity to facilitate efficient problem-solving. For instance, in supply chain management, identifying patterns in inventory fluctuations, delivery delays, or demand variations can highlight bottlenecks and trigger corrective actions. In customer relationship management, recognizing recurring buying patterns allows businesses to tailor marketing strategies, anticipate future needs, and enhance customer satisfaction. The ability to recognize patterns in data is the key to deriving value and insights from vast amounts of information, a crucial component of "griks" approaches. Furthermore, in areas like fraud detection, pattern recognition algorithms can identify unusual transaction patterns indicative of fraudulent activity. These examples demonstrate the broad applicability of pattern recognition across diverse fields. The core principle in each case centers on the ability to extract actionable information from intricate datasets, a capability crucial to "griks" methods.
In summary, pattern recognition is not merely a component but a core strength of "griks." Its ability to extract meaning from complex data empowers predictive modeling, facilitates system optimization, and allows for effective responses to evolving situations. While challenges remain in identifying complex patterns within noisy or incomplete data sets, the significance of pattern recognition in "griks" is undeniable. Its ability to unveil hidden connections and establish actionable insights underscores its critical role in the evolving landscape of complex systems analysis and problem-solving.
Frequently Asked Questions about "griks"
This section addresses common inquiries regarding the "griks" methodology. These questions and answers aim to clarify key concepts and applications of this analytical approach.
Question 1: What is "griks" in the context of complex systems analysis?
The term "griks" describes a comprehensive approach to analyzing and modeling complex systems. This framework encompasses data structuring, algorithmic design, network analysis, predictive modeling, system optimization, and pattern recognition. It emphasizes the interconnectedness of various elements within a system and utilizes these interdependencies to predict future behavior and optimize system performance.
Question 2: How does "griks" differ from other analytical methodologies?
"Griks" distinguishes itself by its holistic perspective. Unlike isolated analyses, "griks" considers the intricate relationships between components of a system. It leverages network analysis to understand the intricate web of interactions, providing a broader, more interconnected understanding of the system's dynamics than more traditional approaches.
Question 3: What are the practical applications of "griks" in real-world scenarios?
Applications span diverse fields. In supply chain management, "griks" can help identify bottlenecks and optimize logistics. Financial institutions can leverage it to assess market risks and forecast future trends. Epidemiologists can use "griks" models to predict disease outbreaks. Furthermore, urban planners can utilize "griks" insights to model and optimize urban environments.
Question 4: What data types are suitable for "griks" analysis?
"Griks" analysis thrives on diverse data types. Structured data, including relational databases, is a crucial component. However, "griks" methods also effectively incorporate unstructured data, such as text, images, or social media content, by transforming it into a usable format for analysis. The specific data requirements vary based on the nature of the system being analyzed.
Question 5: What are the limitations or challenges associated with "griks" methodologies?
The effectiveness of "griks" relies heavily on the quality and availability of data. Incomplete or inaccurate data can yield unreliable results. Furthermore, the complexity of some systems can make precise modeling challenging. Computational resources and expert interpretation are often required for "griks" analysis to provide meaningful insight.
In conclusion, "griks" presents a robust approach to complex systems analysis. While challenges exist, the potential for valuable insights and impactful applications makes it a promising methodology. The successful implementation requires careful consideration of data quality, computational resources, and expert interpretation.
Next, we delve into the detailed methodologies and specific techniques associated with the "griks" framework.
Conclusion
The exploration of "griks" reveals a comprehensive analytical framework for understanding complex systems. Key elements highlighted include data structuring, algorithmic design, network analysis, predictive modeling, system optimization, and pattern recognition. The framework emphasizes the interconnectedness of system components, allowing for a deeper understanding of their dynamic interactions and emergent behaviors. The methodology draws upon diverse data types, from structured relational data to unstructured information, and utilizes sophisticated techniques for processing and interpreting vast datasets. The ability to identify patterns and predict future outcomes within these complex systems presents significant potential for informed decision-making and strategic interventions.
Further research and development within the "griks" framework are essential to address its inherent complexities. Challenges remain in managing massive datasets, ensuring data accuracy, and refining predictive models. However, the potential of "griks" to unlock insights within diverse fields, from finance to healthcare, warrants continued exploration. The insights gained can guide proactive strategies, optimize resource allocation, and mitigate risks associated with intricate system dynamics. This profound understanding holds the potential to transform the way we approach complex problem-solving in the future.
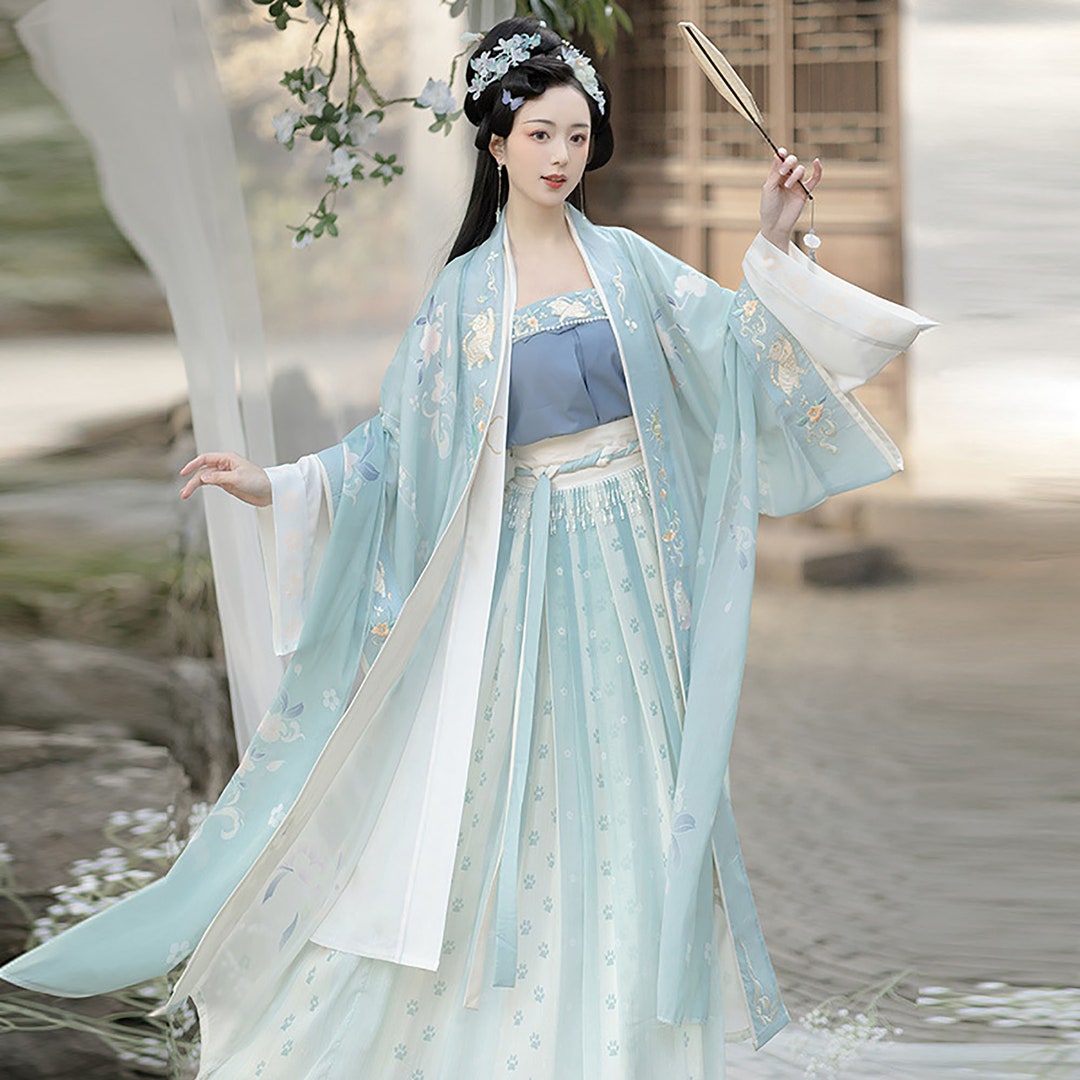
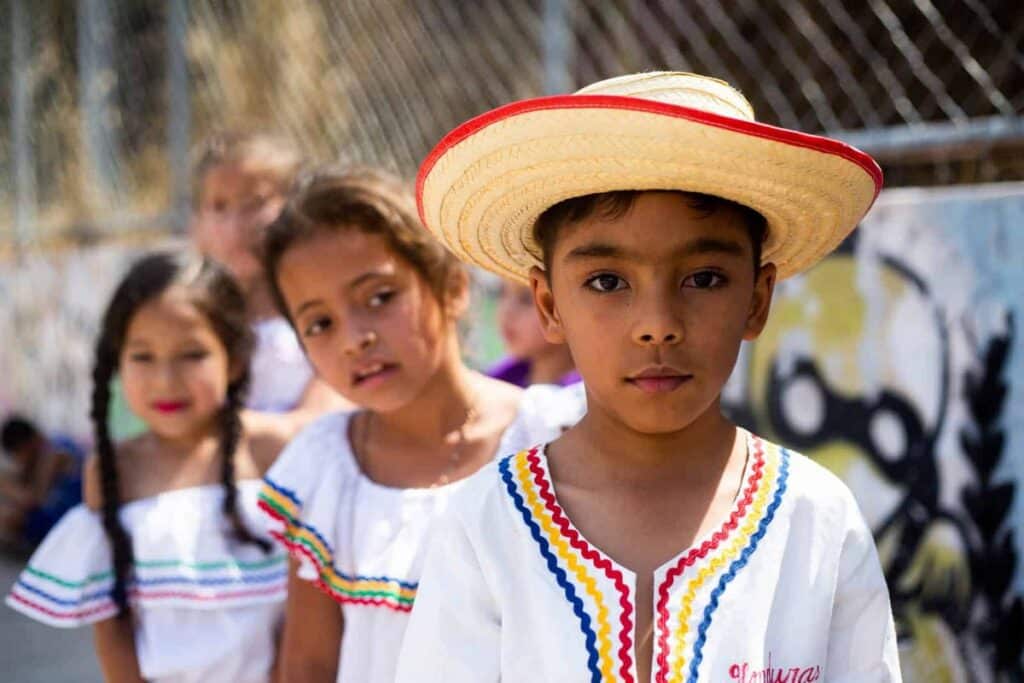
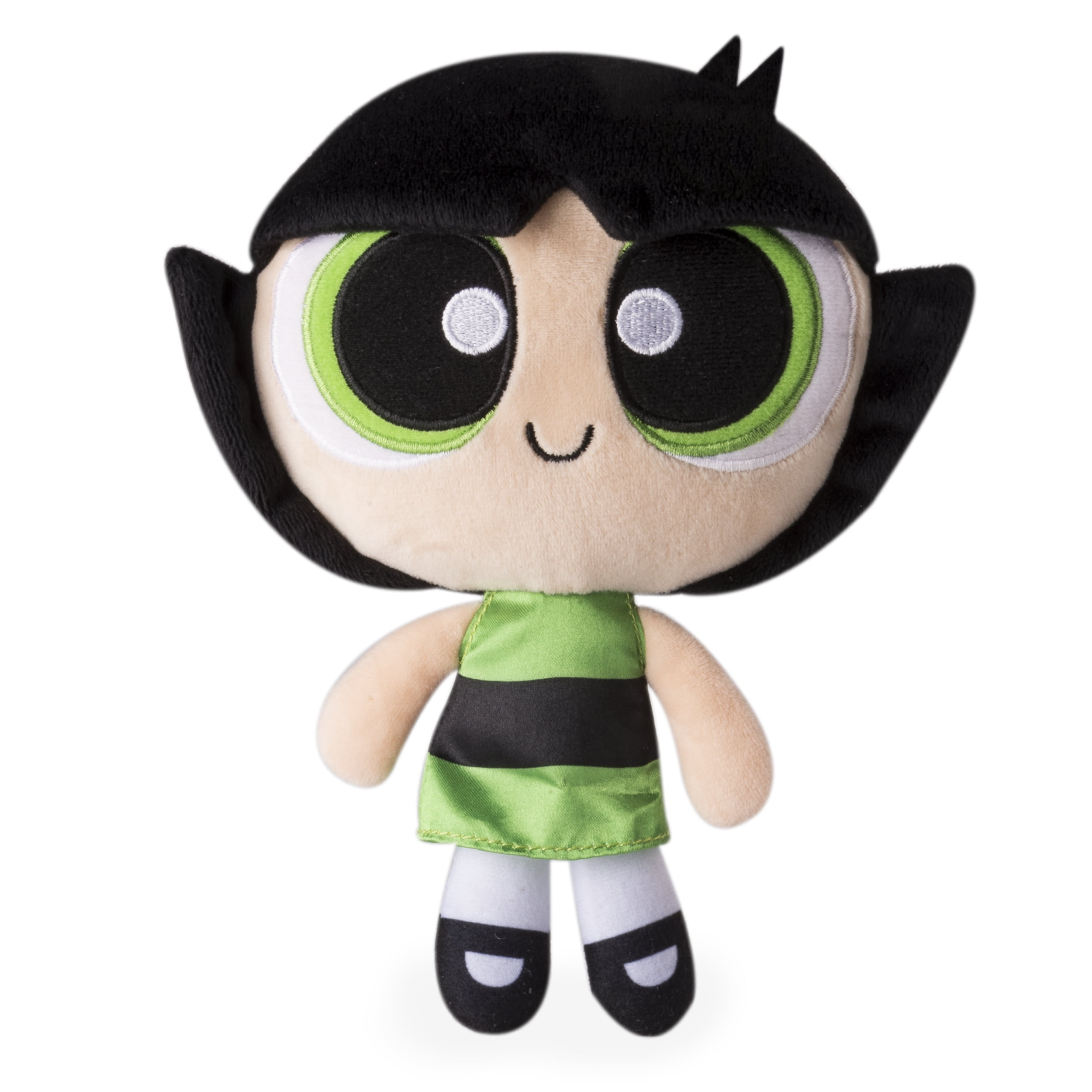