What are the implications of advanced conversational AI? How does this technology impact communication and information access?
Advanced conversational agents, characterized by their ability to understand and respond to complex, nuanced human language, represent a significant development in artificial intelligence. These systems are designed to engage in interactive dialogue, providing information, generating text, and performing tasks. Examples include virtual assistants, customer service bots, and language translation tools. The sophistication of these systems is increasing rapidly, enabling them to handle increasingly complex and multifaceted requests.
The importance of these systems lies in their potential to streamline communication, enhance accessibility, and revolutionize various sectors. Improved customer service, efficient information retrieval, and personalized learning experiences are among the tangible benefits. Historical context reveals a progression from rudimentary rule-based systems to more sophisticated machine learning models, demonstrating a growing capacity to mimic and even augment human conversation. The continued evolution of these tools promises further advancements in fields ranging from education to healthcare.
Advanced Conversational AI
Understanding and interacting with advanced conversational AI, often referred to as a "chatpot," requires consideration of several key dimensions. These aspects, encompassing technological capabilities, practical applications, and ethical implications, are crucial for a thorough understanding.
- Natural Language Processing
- Machine Learning
- Dialogue Management
- Contextual Understanding
- Task Fulfillment
- Data Security
Advanced conversational AI systems rely on sophisticated natural language processing techniques to interpret human language. Machine learning algorithms enable these systems to learn and improve their responses over time, leading to more nuanced and accurate interactions. Effective dialogue management ensures coherent and logical conversations, while contextual understanding allows for tailored responses. Task fulfillment capabilities range from information retrieval to completing simple commands. However, the safeguarding of user data is paramount, as conversational AI systems frequently interact with sensitive information. These systems have significant implications in fields such as customer service, information access, and automated tasks, demonstrating potential benefits while requiring careful consideration of security and ethical implications.
1. Natural Language Processing
Natural Language Processing (NLP) forms the bedrock of advanced conversational agents, frequently referred to as "chatbots." NLP empowers these systems to understand, interpret, and generate human language. Without robust NLP capabilities, a system cannot engage in meaningful dialogue. The ability to parse sentences, identify entities, discern sentiment, and translate intent is fundamental to a chatbot's functionality. Real-world examples include virtual assistants capable of scheduling appointments, answering questions, and managing tasks by deciphering user requests and transforming them into actionable steps.
The significance of NLP in chatbots extends beyond basic comprehension. Sophisticated NLP models analyze the nuances of language, including sarcasm, humor, and contextual subtleties. This allows for a more human-like interaction. For instance, a chatbot designed for customer service can recognize frustration in a user's query and respond with appropriate empathy. This nuanced understanding of language enables the development of chatbots that are not simply transactional but also supportive and helpful. Moreover, the ability to extract information and discern intent from complex sentences and phrases is critical for efficient task completion and information retrieval.
In conclusion, Natural Language Processing is an indispensable component of advanced conversational agents. Its role in enabling sophisticated understanding and generation of human language is critical for practical applications. While challenges remain in achieving perfect language understanding, ongoing advancements in NLP continue to enhance the capabilities of chatbots, leading to more natural and effective interactions in diverse contexts.
2. Machine Learning
Machine learning is a cornerstone of modern conversational agents, often referred to as "chatbots." It underpins the ability of these systems to learn from data, adapting to user interactions and improving their performance over time. This learning process is crucial for achieving sophisticated conversational capabilities.
- Data Training and Learning
Chatbots are trained on vast datasets of text and conversations. Machine learning algorithms analyze this data to identify patterns, extract meaning, and establish correlations between user inputs and appropriate responses. This training process allows the system to understand the nuances of human language and context, thereby enabling more natural and accurate interactions. Examples include training datasets of customer service dialogues, online forums, or social media posts.
- Adaptation and Improvement
Machine learning enables chatbots to adapt to evolving user behavior and language. As the system processes more interactions, it progressively adjusts its responses to align with user expectations. This adaptability is exemplified by chatbots that learn to resolve customer queries or provide specific product recommendations with increasing accuracy over time.
- Contextual Understanding
Machine learning algorithms play a pivotal role in enhancing a chatbot's ability to understand context. By analyzing the flow of a conversation, the system can comprehend the user's intent and maintain relevant responses throughout the dialogue. This contextual awareness is essential for managing complex interactions that often span several turns or requests.
- Natural Language Generation
Machine learning facilitates the generation of human-like text by chatbots. Training models on diverse texts enables the system to create coherent and relevant responses based on the context of the conversation. This leads to more fluid and engaging interactions, enabling the chatbot to provide not only information but also to communicate in a conversational and user-friendly manner.
These facets collectively demonstrate the crucial role of machine learning in enabling advanced conversational agents. By continuously learning and adapting to user interactions, chatbots powered by machine learning can provide more efficient, effective, and user-centric services. The ability to process vast datasets, interpret user intent, and generate appropriate responses distinguishes these systems from earlier, rule-based approaches and points to the ongoing potential of this technology.
3. Dialogue Management
Dialogue management is a critical component of advanced conversational agents, often referred to as "chatbots." It acts as the orchestrator of interactions, ensuring a coherent and effective exchange between the system and the user. Without robust dialogue management, even the most sophisticated natural language processing and machine learning capabilities can result in disjointed or illogical conversations. Effective dialogue management structures the conversation flow, guiding the exchange toward desired outcomes while maintaining user engagement.
A well-designed dialogue management system employs strategies to maintain context, handle user input variations, and progress through predefined stages. This structure facilitates efficient information retrieval, task completion, or resolution of queries. Real-world examples include customer service chatbots that transition through different stages of a support interaction, collecting information, addressing issues, and guiding users toward solutions. Similarly, in educational settings, chatbots utilizing dialogue management can present lessons, provide feedback, and adapt to individual learning styles, thereby enhancing knowledge acquisition. The seamless transition between these stages, managed by the dialogue management system, is crucial for user satisfaction and the effective achievement of predefined goals.
Understanding dialogue management's role within a chatbot is vital for optimizing performance and user experience. A poorly designed dialogue management system can lead to frustrating user interactions, hindering the successful completion of tasks or the efficient delivery of information. Challenges include adapting to unpredictable user input, handling interruptions or irrelevant information, and maintaining context across multiple turns in a conversation. However, robust dialogue management systems, with their ability to structure and maintain conversational flow, significantly enhance the usability and effectiveness of advanced conversational agents in diverse applications. This emphasizes the importance of careful planning and design when implementing dialogue management strategies for chatbots, thereby ensuring the system effectively serves its intended purpose.
4. Contextual Understanding
Contextual understanding is integral to the functionality and effectiveness of advanced conversational agents, frequently referred to as "chatbots." The ability to discern context within a conversation is crucial for generating appropriate and relevant responses. A chatbot lacking contextual understanding will likely struggle to comprehend user intent and provide inaccurate or irrelevant information. This deficiency can lead to poor user experience, hindering the achievement of desired outcomes. For example, a customer service chatbot might misinterpret a complaint due to a lack of contextual awareness, failing to identify the underlying issue and escalating the problem.
Contextual understanding operates across multiple layers. Initially, the system needs to recognize the overall topic or subject of the conversation. Furthermore, the chatbot must grasp the current state of the dialogue, taking into account previous user input and responses. This understanding encompasses recognizing the intent behind user requests and anticipating subsequent needs. A robust contextual understanding enables the chatbot to provide comprehensive, coherent responses, moving beyond simple keyword matching and into a more nuanced comprehension of the conversation's flow. This depth of understanding is evident in chatbots capable of maintaining a meaningful conversation across several turns, resolving complex issues, and providing tailored recommendations.
The significance of contextual understanding extends to numerous practical applications. In customer service, a chatbot demonstrating this understanding can provide tailored solutions, efficiently resolving queries and improving customer satisfaction. In e-commerce, contextual understanding enables personalized product recommendations and targeted marketing campaigns, thereby enhancing user engagement and driving sales. Similarly, educational chatbots leveraging contextual understanding can adapt to individual learning styles, providing personalized support and improving knowledge retention. Consequently, the effectiveness of these applications directly correlates with the degree of contextual awareness embedded within the chatbot system. While challenges remain in ensuring complete and consistent contextual understanding, continued advancements in artificial intelligence and machine learning promise further enhancements in this critical area, leading to more sophisticated and user-friendly conversational agents.
5. Task Fulfillment
Task fulfillment is a critical aspect of advanced conversational agents, often referred to as chatbots. It represents the ability of these systems to complete specific actions or tasks based on user requests. The connection between task fulfillment and a chatbot lies in its practical application: a chatbot's effectiveness hinges on its capacity to execute desired actions. This capability is a key determinant of user satisfaction and the overall utility of the system.
The importance of task fulfillment as a component of a chatbot is underscored by its diverse applications. For instance, a chatbot used for customer service might need to book appointments, retrieve order details, or process refunds. Similarly, in an educational setting, a chatbot might be tasked with scheduling tutoring sessions, delivering course materials, or grading assignments. Each of these tasks, when successfully completed, contributes to the chatbot's overall effectiveness and user satisfaction. Successfully fulfilling tasks relies heavily on the chatbot's ability to interpret user requests, access relevant information, and execute the requested action. Accurate interpretation prevents misunderstandings, while efficient access to information ensures timely and effective task completion. The complexity of the tasks varies considerably, from basic inquiries to intricate processes. The ability to handle a wider array of tasks signifies enhanced capabilities for the chatbot.
In summary, task fulfillment is not merely a feature of a chatbot; it is central to its purpose and value. The success of a conversational agent is directly tied to its ability to effectively and efficiently execute the tasks assigned to it. This capability is critical for numerous applications, from customer service and education to e-commerce and healthcare. Challenges in task fulfillment include ensuring accuracy, handling variations in user language, and managing complex processes. Overcoming these obstacles is crucial for realizing the full potential of chatbots and their ability to streamline processes and improve user experience.
6. Data Security
Data security is paramount in the context of advanced conversational agents, frequently referred to as chatbots. These systems often collect, process, and store vast amounts of user data, making robust security measures essential. Compromised data security can lead to significant repercussions, including privacy violations, financial losses, and reputational damage. A compromised chatbot can expose sensitive information about users, potentially leading to identity theft or fraudulent activities. For instance, a customer service chatbot handling financial transactions must maintain high levels of security to prevent unauthorized access and data breaches. The importance of data protection extends to educational chatbots handling student records, and medical chatbots handling sensitive patient information.
Protecting user data within chatbots requires a multi-layered approach. Data encryption during transmission and storage is critical. Access controls and authentication mechanisms are essential to restrict data access to authorized personnel. Regular security audits and vulnerability assessments can identify and mitigate potential security risks. Implementing robust data governance policies and adhering to industry regulations, such as HIPAA or GDPR, are critical components of a comprehensive security strategy. Examples include employing end-to-end encryption for sensitive conversations, using multi-factor authentication to verify user identities, and employing secure infrastructure for storing and processing data. Strict data anonymization procedures are also vital for protecting privacy when using large training datasets to train chatbots.
Understanding the critical link between data security and advanced conversational agents is essential for both developers and users. Robust data security practices are not just technical necessities; they are ethical responsibilities. Without strong security measures, users may be reluctant to interact with chatbots, fearing potential risks to their personal information. Ultimately, a balance must be struck between developing innovative conversational AI and upholding data security protocols. This requires continuous advancements in security technologies, proactive risk management strategies, and a commitment to user privacy throughout the entire lifecycle of a chatbot's development, operation, and data usage.
Frequently Asked Questions about Advanced Conversational Agents
This section addresses common queries concerning advanced conversational agents, often referred to as chatbots. These questions cover key aspects of functionality, limitations, and ethical considerations.
Question 1: What are the limitations of advanced conversational agents?
Advanced conversational agents, while exhibiting impressive capabilities, are not without limitations. These systems, reliant on vast datasets and complex algorithms, may sometimes struggle with nuanced or ambiguous language. Contextual understanding can be imperfect, leading to inappropriate or inaccurate responses. Furthermore, the ability to perform tasks outside pre-programmed parameters is limited. These agents excel at executing defined tasks but may lack adaptability in unforeseen situations. The accuracy of responses depends heavily on the quality and comprehensiveness of the training data. Finally, the potential for biases embedded within the training data can manifest in the output, requiring ongoing vigilance and refinement to mitigate these biases.
Question 2: How secure are the data interactions with these systems?
Data security is paramount. Advanced conversational agents frequently handle sensitive information. Robust encryption protocols, access controls, and regular security assessments are crucial. However, security remains a continuous concern. The security of these systems hinges on the strength of the underlying security infrastructure and the vigilance of security protocols. Regular audits and adherence to data privacy regulations are vital for ensuring the security of user data and mitigating potential risks.
Question 3: What role does machine learning play in conversational agents?
Machine learning is fundamental to the functionality of advanced conversational agents. Algorithms are trained on vast datasets, enabling the system to learn patterns in language and improve response accuracy over time. This continuous learning process allows for adaptation to evolving user language and expectations, enhancing performance and achieving increasingly natural interactions. However, the quality of training data directly impacts the accuracy and appropriateness of responses generated.
Question 4: What are the ethical considerations surrounding the use of these agents?
Ethical considerations are crucial. The potential for biased responses, misuse of information, and the impact on human interaction require careful attention. Developing these systems demands mindful consideration of fairness, transparency, and potential consequences of deploying these technologies in various contexts. Responsible development and deployment are critical to harnessing the potential of these tools while mitigating negative impacts.
Question 5: How does contextual understanding impact interactions with conversational agents?
Contextual understanding is essential for sophisticated conversational interactions. By considering previous exchanges, the system can maintain coherence, generating relevant and meaningful responses. Accurate context interpretation ensures appropriate behavior throughout the interaction, allowing for more fluid and helpful exchanges. However, maintaining context across extensive conversations can present challenges, highlighting the need for robust models to effectively track and utilize conversational history.
These FAQs offer a concise overview of frequently raised concerns regarding advanced conversational agents. Understanding these limitations, security issues, and ethical considerations are essential for navigating the practical implications and applications of these systems.
Moving forward, examining specific use cases of these technologies, such as in customer service or education, would provide additional insight.
Conclusion
This exploration of advanced conversational agents, often termed "chatbots," reveals a multifaceted technology. Key components, including natural language processing, machine learning, dialogue management, and contextual understanding, underpin the functionality of these systems. Task fulfillment capabilities, however, are contingent upon the robustness of these underlying processes. Critically, data security considerations are paramount, demanding robust protocols and ethical considerations to mitigate potential risks. The interplay of these elements shapes the utility and limitations of advanced conversational agents. The exploration underscores both the promise and the challenges inherent in this burgeoning field.
The future trajectory of "chatbots" hinges on addressing existing limitations and proactively confronting ethical considerations. Continued development must focus on improving contextual understanding, enhancing task fulfillment capabilities, and bolstering data security protocols. This demands a collaborative effort among researchers, developers, and users to ensure responsible innovation and ethical deployment. The potential societal impact of these systems is substantial, and proactive engagement with the challenges they present is essential to realizing their positive applications while mitigating potential risks.
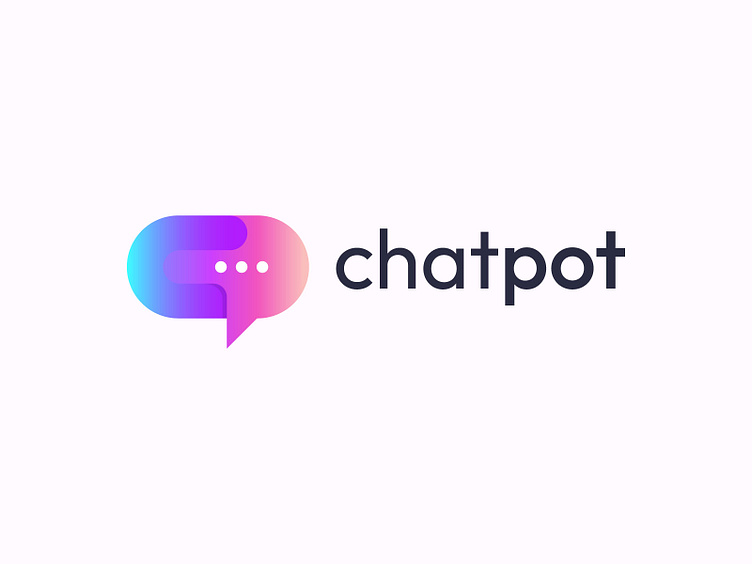
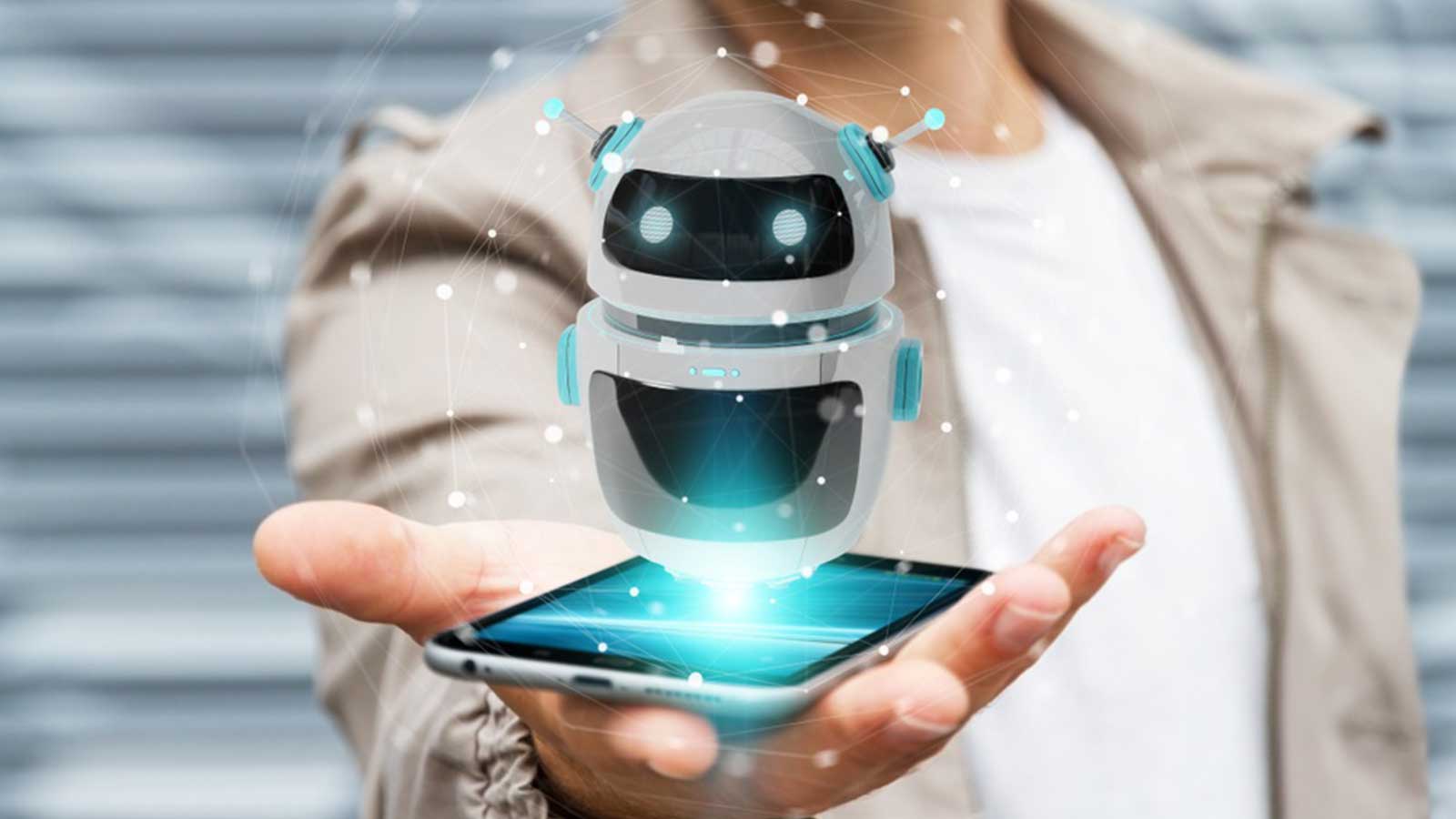
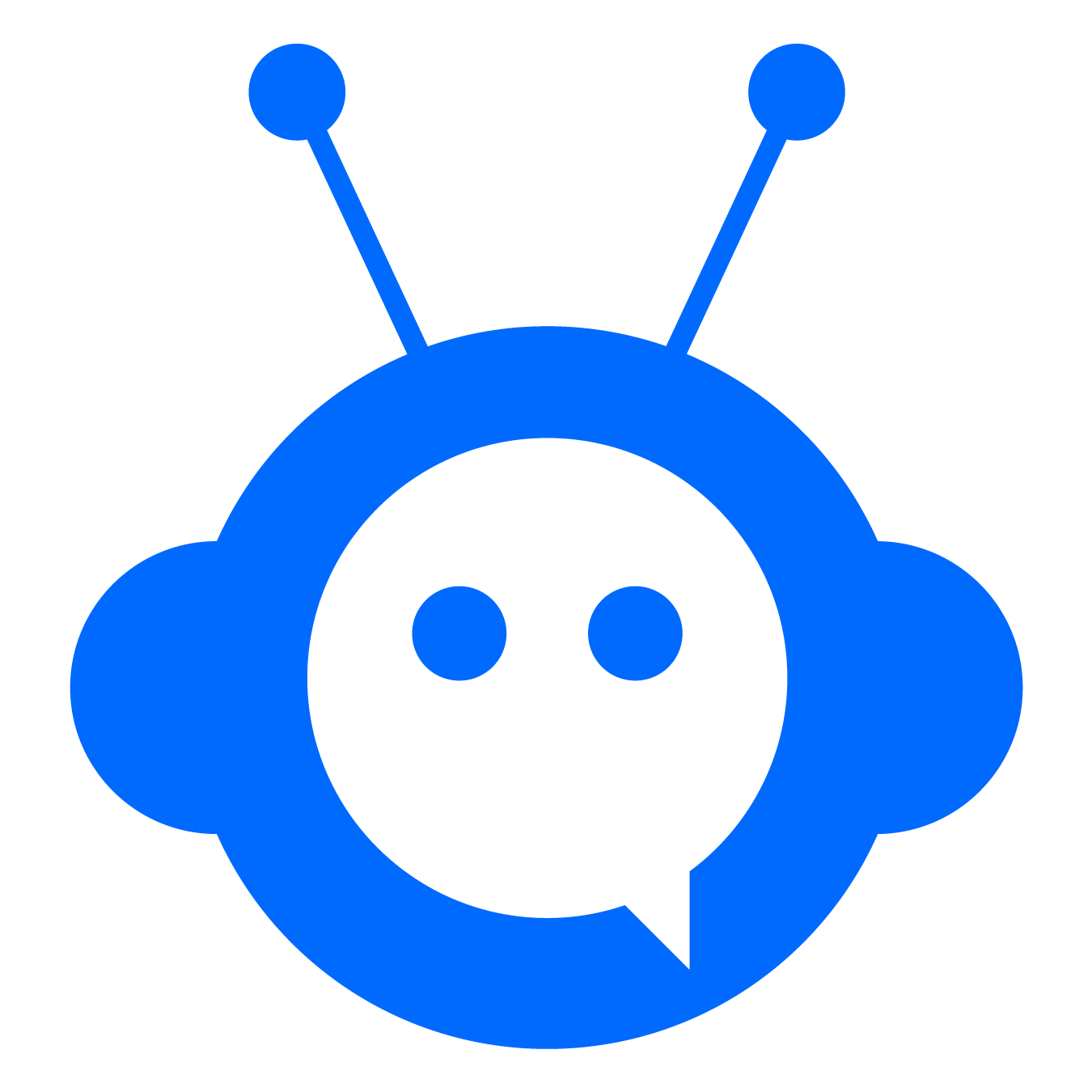